Tag: fair use
UC Berkeley Library to Copyright Office: Protect fair uses in AI training for research and education
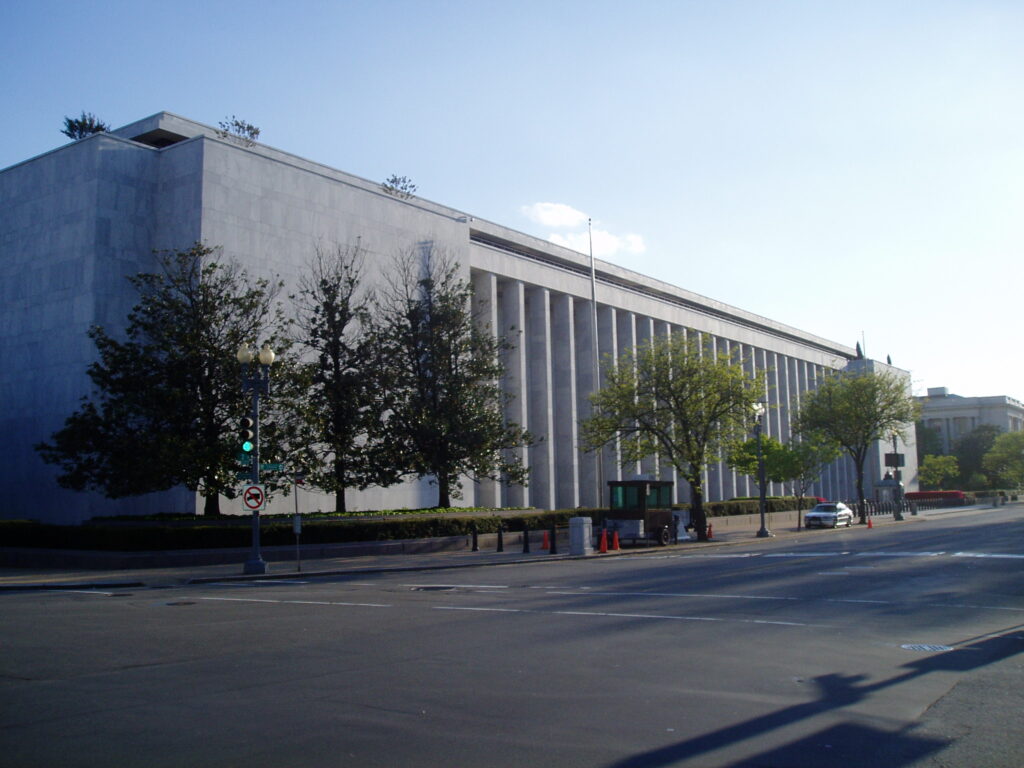
We are pleased to share the UC Berkeley Library’s response to the U.S. Copyright Office’s Notice of Inquiry regarding artificial intelligence and copyright. Our response addresses the essential fair use right relied upon by UC Berkeley scholars in undertaking groundbreaking research, and the need to preserve access to the underlying copyright-protected content so that scholars using AI systems can conduct research inquiries.
In this blog post, we explain what the Copyright Office is studying, and why it was important for the Library to make scholars’ voices heard.
What the Copyright Office is studying and why
Loosely speaking, the Copyright Office wants to understand how to set policy for copyright issues raised by artificial intelligence (“AI”) systems.
Over the last year, AI systems and the rapid growth of their capabilities have attracted significant attention. One type of AI, referred to as “generative AI”, is capable of producing outputs such as text, images, video, or audio (including emulating a human voice) that would be considered copyrightable if created by a human author. These systems include, for instance, the chatbot ChatGPT, and text-to-image generators like DALL·E, Midjourney, and Stable Diffusion. A user can prompt ChatGPT to write a short story that features a duck and a frog who are best friends, or prompt DALL·E to create an abstract image in the style of a Jackson Pollock painting. Generative AI systems are relevant to and impact many educational activities on a campus like UC Berkeley, but (at least to date) have not been the key facilitator of campus research methodologies.
Instead, in the context of research, scholars have been relying on AI systems to support a set of research methodologies referred to as “text and data mining” (or TDM). TDM utilizes computational tools, algorithms, and automated techniques to extract revelatory information from large sets of unstructured or thinly-structured digital content. Imagine you have a book like “Pride and Prejudice.” There are nearly infinite volumes of information stored inside that book, depending on your scholarly inquiry, such as how many female vs. male characters there are, what types of words the female characters use as opposed to the male characters, what types of behaviors the female characters display relative to the males, etc. TDM allows researchers to identify and analyze patterns, trends, and relationships across volumes of data that would otherwise be impossible to sift through on a close examination of one book or item at a time.
Not all TDM research methodologies necessitate the usage of AI systems to extract this information. For instance, as in the “Pride and Prejudice” example above, sometimes TDM can be performed by developing algorithms to detect the frequency of certain words within a corpus, or to parse sentiments based on the proximity of various words to each other. In other cases, though, scholars must employ machine learning techniques to train AI models before the models can make a variety of assessments.
Here is an illustration of the distinction: Imagine a scholar wishes to assess the prevalence with which 20th century fiction authors write about notions of happiness. The scholar likely would compile a corpus of thousands or tens of thousands of works of fiction, and then run a search algorithm across the corpus to detect the occurrence or frequency of words like “happiness,” “joy,” “mirth,” “contentment,” and synonyms and variations thereof. But if a scholar instead wanted to establish the presence of fictional characters who embody or display characteristics of being happy, the scholar would need to employ discriminative modeling (a classification and regression technique) that can train AI to recognize the appearance of happiness by looking for recurring indicia of character psychology, behavior, attitude, conversational tone, demeanor, appearance, and more. This is not using a generative AI system to create new outputs, but rather training a non-generative AI system to predict or detect existing content. And to undertake this type of non-generative AI training, a scholar would need to use a large volume of often copyright-protected works.
The Copyright Office is studying both of these kinds of AI systems—that is, both generative AI and non-generative AI. They are asking a variety of questions in response to having been contacted by stakeholders across sectors and industries with diverse views about how AI systems should be regulated. Some of the concerns expressed by stakeholders include:
- Who is the “author” of generative AI outputs?
- Should people whose voices or images are used to train generative AI systems have a say in how their voices or images are used?
- Should the creator of an AI system (whether generative or non-generative) need permission from copyright holders to use copyright-protected materials in training the AI to predict and detect things?
- Should copyright owners get to opt out of having their content used to train AI? Should ethics be considered within copyright regulation?
Several of these questions are already the subject of pending litigation. While these questions are being explored by the courts, the Copyright Office wants to understand the entire landscape better as it considers what kinds of AI copyright regulations to enact.
The copyright law and policy landscape underpinning the use of AI models is complex, and whatever regulatory decisions that the Copyright Office makes will bear ramifications for global enterprise, innovation, and trade. The Copyright Office’s inquiry thus raises significant and timely legal questions, many of which we are only beginning to understand.
For these reasons, the Library has taken a cautious and narrow approach in its response to the inquiry: we address only two key principles known about fair use and licensing, as these issues bear upon the nonprofit education, research, and scholarship undertaken by scholars who rely on (typically non-generative) AI models. In brief, the Library wants to ensure that (1) scholars’ voices, and that of the academic libraries who support them, are heard to preserve fair use in training AI, and that (2) copyright-protected content remains available for AI training to support nonprofit education and research.
Why the study matters for fair use
Previous court cases like Authors Guild v. HathiTrust, Authors Guild v. Google, and A.V. ex rel. Vanderhye v. iParadigms have addressed fair use in the context of TDM and determined that the reproduction of copyrighted works to create and text mine a collection of copyright-protected works is a fair use. These cases further hold that making derived data, results, abstractions, metadata, or analysis from the copyright-protected corpus available to the public is also fair use, as long as the research methodologies or data distribution processes do not re-express the underlying works to the public in a way that could supplant the market for the originals. Performing all of this work is essential for TDM-reliant research studies.
For the same reasons that the TDM process is fair use of copyrighted works, the training of AI tools to do that TDM should also be fair use, in large part because training does not reproduce or communicate the underlying copyrighted works to the public. Here, there is an important distinction to make between training inputs and outputs, in that the overall fair use of generative AI outputs cannot always be predicted in advance: The mechanics of generative models’ operations suggest that there are limited instances in which generative AI outputs could indeed be substantially similar to (and potentially infringing of) the underlying works used for training; this substantial similarity is possible typically only when a training corpus is rife with numerous copies of the same work. However, the training of AI models by using copyright-protected inputs falls squarely within what courts have determined to be a transformative fair use, especially when that training is for nonprofit educational or research purposes. And it is essential to protect the fair use rights of scholars and researchers to make these uses of copyright-protected works when training AI.
Further, were these fair use rights overridden by limiting AI training access to only “safe” materials (like public domain works or works for which training permission has been granted via license), this would exacerbate bias in the nature of research questions able to be studied and the methodologies available to study them, and amplify the views of an unrepresentative set of creators given the limited types of materials available with which to conduct the studies.
Why access to AI training content should be preserved
For the same reasons, it is important that scholars’ ability to access the underlying content to conduct AI training be preserved. The fair use provision of the Copyright Act does not afford copyright owners a right to opt out of allowing other people to use their works for good reason: if content creators were able to opt out, the provision for fair use would be undermined, and little content would be available to build upon for the advancement of science and the useful arts. Accordingly, to the extent that the Copyright Office is considering creating a regulatory right for creators to opt out of having their works included in AI training, it is paramount that such opt-out provision not be extended to any AI training or activities that constitute fair use, particularly in the nonprofit educational and research contexts.
AI training opt-outs would be a particular threat for research and education because fair use in these contexts is already becoming an out-of-reach luxury even for the wealthiest institutions. Academic libraries are forced to pay significant sums each year to try to preserve fair use rights for campus scholars through the database and electronic content license agreements that libraries sign. In the U.S., the prospect of “contractual override” means that, although fair use is statutorily provided for, private parties (like publishers) may “contract around” fair use by requiring libraries to negotiate for otherwise lawful activities (such as conducting TDM or training AI for research), and often to pay additional fees for the right to conduct these lawful activities on top of the cost of licensing the content, itself. When such costs are beyond institutional reach, the publisher or vendor may then offer similar contractual terms directly to research teams, who may feel obliged to agree in order to get access to the content they need. Vendors may charge tens or even hundreds of thousands of dollars for this type of access.
This “pay-to-play” landscape of charging institutions for the opportunity to rely on existing statutory rights is particularly detrimental for TDM research methodologies, because TDM research often requires use of massive datasets with works from many publishers, including copyright owners that cannot be identified or who are unwilling to grant licenses. If the Copyright Office were to enable rightsholders to opt-out of having their works fairly used for training AI, then academic institutions and scholars would face even greater hurdles in licensing content for research purposes.
First, it would be operationally difficult for academic publishers and content aggregators to amass and license the “leftover” body of copyrighted works that remain eligible for AI training. Costs associated with publishers’ efforts in compiling “AI-training-eligible” content would be passed along as additional fees charged to academic libraries. In addition, rightsholders might opt out of allowing their work to be used for AI training fair uses, and then turn around and charge AI usage fees to scholars (or libraries)—essentially licensing back fair uses for research. These scenarios would impede scholarship by or for research teams who lack grant or institutional funds to cover these additional expenses; penalize research in or about underfunded disciplines or geographical regions; and result in bias as to the topics and regions studied.
Scholars need to be able to utilize existing knowledge resources to create new knowledge goods. Congress and the Copyright Office clearly understand the importance of facilitating access and usage rights, having implemented the statutory fair use provision without any exclusions or opt-outs. This status quo should be preserved for fair use AI training—and particularly in the nonprofit educational or research contexts.
Our office is here to help
No matter what happens with the Copyright Office’s inquiry and any regulations that ultimately may be established, the UCB Library’s Office of Scholarly Communication Services is here to help you. We are a team of copyright law and information policy (licensing, privacy, and ethics) experts who help UC Berkeley scholars navigate legal, ethical, and policy considerations in utilizing resources in their research and teaching. And we are national and international leaders in supporting TDM research—offering online tools, trainings, and individual consultations to support your scholarship. Please feel free to reach out to us with any questions at schol-comm@berkeley.edu.
Workshop Reminder—Copyright & Fair Use for Digital Projects
Workshop Date/Time: Tuesday, November 8, 2022, 11:00am–12:30pm
RSVP for Zoom link
Please sign up today and join us on November 8.
Upcoming workshop reminder: Copyright & Fair Use for Digital Projects
We just wrapped up three publishing workshops last week, but there’s more in store. Check out the details below and sign up for the next one offered by the Office of Scholarly Communication Services. See you there!
Copyright and Fair Use for Digital Projects
November 10, 2021
11:00am–12:30pm
RSVP
This online training will help you navigate the copyright, fair use, and usage rights of including third-party content in your digital project. Whether you seek to embed video from other sources for analysis, post material you scanned from a visit to the archives, add images, upload documents, or more, understanding the basics of copyright and discovering a workflow for answering copyright-related digital scholarship questions will make you more confident in your project. We will also provide an overview of your intellectual property rights as a creator and ways to license your own work.
Workshop: Copyright in Course Design and Digital Learning Environments
The Library’s Office of Scholarly Communication Services is hosting an online workshop on July 9, from 10-11:30 on copyright, fair use, and contracts issues that arise in online course development.
Copyright in Course Design and Digital Learning Environments
Workshop: Copyright and Fair Use for Digital Projects
Copyright and Fair Use for Digital Projects
Wednesday, March 14th, 11:10-12:40pm
D-Lab, 350 Barrows Hall
This training will help you navigate the copyright, fair use, and usage rights of including third-party content in your digital project. Whether you seek to embed video from other sources for analysis, post material you scanned from a visit to the archives, add images, upload documents, or more, understanding the basics of copyright and discovering a workflow for answering copyright-related digital scholarship questions will make you more confident in your publication. We will also provide an overview of your intellectual property rights as a creator and ways to license your own work. Register at bit.ly/dp-berk
Upcoming Workshops in this Series 2017-2018:
- Omeka for Digital Collections and Exhibits
- By Design: Graphics & Images Basics
- The Long Haul: Best Practices for Making Your Digital Project Last
Please see bit.ly/dp-berk for details.
Participate in an Affordable Course Content Pilot Program!
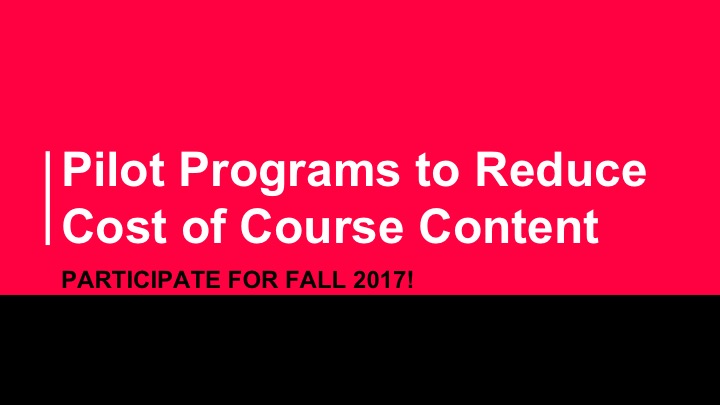
Dear UC Berkeley faculty and lecturers,
We can help you make your assigned readings and textbooks more affordable to students. The Library and the Center for Teaching & Learning have launched two pilot programs for Fall 2017, for which your participation can save students potentially hundreds of dollars each.
-
The first pilot service aims to reduce the cost of your print course packs through Library-assisted syllabus processing. We will locate copies of open, free, or Library-licensed versions of your assigned readings so the overall price of the print course pack or course reader is reduced.
-
The second service provides you with grants to either use, adapt, or develop open or library-licensed electronic textbooks and course materials. This can help save students the cost of purchasing expensive textbooks.
Please fill out this brief form if you are interested in participating in one or both pilots (described more fully below), and we will contact you soon with details.
______________________________
Pilot 1 (Course Packs): Do you assign your students a print course pack for purchase? We can help reduce the cost of that print course pack.
With the first piloted service, the Library will process your syllabus for you and search for your required readings to locate copies of open, free, or Library-licensed versions of assigned readings.
-
If open, free, or Library-licensed versions are available, we will give you links or PDFs to post to bCourses at no cost to your students, reducing any remaining readings that a student would have purchased as part of a print course pack.
-
We will also provide guidance to you for making fair use decisions–further reducing the cost of course packs, because we can help you limit instances in which a third party copy center would need to secure copyright clearance for assigned readings.
______________________________
Pilot 2 (Grants): If you assign textbooks or other books, will you let us pay you from $500 up to $5,000 to switch to an electronic version of that book or to an equivalent eBook or combination of books? Or will you let us help you in adopting, adapting, or designing your own open and electronic course materials?
The Library and the Center for Teaching and Learning are offering grants and programmatic support to instructors to enable you to link to open or Library-licensed electronic textbooks or other eBooks–or even to design your own.
-
The grants range in value from $500 (e.g. for switching one required print book to a Library-licensed electronic book that can be linked to in bCourses) all the way up to $5,000 to receive programmatic support to design your own open & electronic course materials for students so they don’t have to purchase expensive textbooks.
-
The Center for Teaching & Learning and the Library can also help you find campus support to update any other attendant PowerPoints, assignments, or materials that need alteration following a change in assigned books or textbooks.
If you have any questions, please contact the Library’s Scholarly Communication Officer, Rachael Samberg: rsamberg@berkeley.edu. You can also find out more about affordable course content in our Guide to Open, Free, & Affordable Course Materials.
What is Fair Use?
Fair Use is particularly important in academic settings where students, faculty, and researchers are able to legally incorporate copyrighted materials, without permission from the author (but with appropriate attribution, of course) in slide shows, book reviews, and classroom lectures.
To learn more about when Fair Use allows you to use copyrighted material without permission from the copyright holder, check out:
-
Code of Best Practices in Fair Use (from the Association of Research Libraries)
-
Copyright: Fair Use (from the University of California)
-
Fair Use Checklist (from Columbia University Libraries)
-
Fair Use Evaluator (from the American Libraries Association Office of Information Technology Policy)
-
Myths about Fair Use (from Inside Higher Education)
Margaret Phillips, Education-Psychology Library
contact me at mphillip [at] library.berkeley.edu
Reblogged (with permission!) from the Library News blog posting on Fair Use Week.