Author: Bee Lehman
Research Tips: Researching the Author
Many of us, alongside reading poetry and novels or travel narratives, want to know about the context in which the author lived. Who were their families? Did they have a specific person of whom they dreamed when they wrote about love? Understanding the author can give us a better understanding of their meaning, their focus, and their world.
Unsurprisingly, I’d recommend starting with what other scholars have written. If those books don’t exist, or I am after a deeper understanding, I would start looking into what I could sketch out about the author and then look for unpublished papers in archival collections or other repositories using search interfaces such as ArchiveGrid or ArchiveFinder.
The Case Study: Angelina Weld Grimké

For the purposes of this exploration, I’m going to look at one of my many favorite poets: Angelina Weld Grimké (Wikipedia). This poet, born in 1880 in Boston, wrote poignant poems about life and love, many of which were published in the 1910s. They died in 1958 in New York City.
Based on that information, I expect Grimké to have possibly written and received letters to and from family and publishers; to maybe have kept account books or diaries; and possibly to appear in or have created family papers.
There are other kinds of sources that might exist for our purposes as well, but I’m sticking to these for now.
Looking for Unpublished Materials
Unpublished material such as letters is usually held by archives; historical societies/museums; estates; or family/friends. The latter two can be a harder reach, but the first two usually try to let researchers know what they hold. With that aim, many archives upload their finding aids (here’s information from Bancroft Library about what a finding aid is) into collective search interfaces like ArchiveGrid and ArchiveFinder. To find additional ways to search archival collections, take a look at the Library Resource Guide History: Locating Archives.
To use either of those two databases, I usually recommend writing out a list of possible permutations of the person’s name. “Angelina Weld Grimké” might written as such, but it also might appear as:
- Angelina Grimké
- Angelina Weld Grimke
- Angelina Grimke
Some English language search interfaces are designed to ignore accents, and some will fail a search if the characters in a name do not precisely mirror whatever a finding aid says.
Searching ArchiveGrid
OCLC runs this platform and, as far as library search interfaces go, I like it well enough. Do be aware that it sits behind a paywall and you must be logged in with your institution to use it.

Once I have the initial search page up, I usually start my search with the full, formal name; in this case (Angelina Weld Grimké) without quotation marks and with the accent over the “e.”
In this case, I got two results, one of which was the “Weld-Grimké family papers.” When I use the link to the University of Michigan’s finding aid, however, I find out that the collection, substantial at 14 linear feet, focuses on Angelina Weld Grimké’s grandparents, one of whom was abolitionist Angelina Grimké.

The second result, relating to scholar and poet Akasha Hull, is actually more on point as Hull wrote about Angelina Weld Grimké. Based on the collection listed in New York Public Library’s finding aid, however, material about Angelina Weld Grimké is likely to be sparse as the “Scope and arrangement” section note that the collection covers a significant number of topics and the “Detailed description” only mentions Grimké once.
At this point, I ran the other permutations of the name and didn’t get any other relevant collections. So, I move on to the next resource.
Using ArchiveFinder
ProQuest runs ArchiveFinder and I am not a big fan of their interface, largely because of the layout. The point and goal of the interface is much the same, but different archives/repositories subscribe and use different interfaces, which means I need to search both for a wider results list.
So, I start with the same name and search for (Angelina Weld Grimké).

The results here are much more directly on topic. I still get the Michigan result for the Weld family papers at the top. The following results, however, are new and include a collection called “Grimké, Angelina Weld” AND “Angelina Weld Grimké papers, 1887-1958” both at Howard. A few of the other collections, including Fisk University’s “Negro collection” appear to hold potentially interesting information.
Reading a Relevant Finding Aid
In deciding which collections to focus on, I read the associated finding aids, focusing first on description and size. For Howard’s “Grimké, Angelina Weld” (finding aid) is 8 linear feet and includes drafts as well as published material.

Given the highly-relevant description, I then skim the rest of the finding aid, which includes folder and box level description. That means Howard hasn’t recorded information about every piece of information in the box, but you can get a great sense of what’s there. For example, the description for Box 38, folder 15:
Series F Notebooks Box 38-15 French vocabulary exercises, writings of prose and poetry, and recipes
suggests that it contains some poetry along with other types of material. That might not give a clear sense of what poetry is in there or how it’s presented, but something of interest is there! Or, I think it will be. My personal, vague, castle-in-the-sky hope is that there will be poems about food scrawled on whatever recipe information is there.
If there are only one or two papers that are of interest, then I’d likely check the archive’s information about rights and reproductions. If they have options for digital copies, I might request the page or two.
In this case, there are hundreds of items associated with the collection. Most archives won’t copy that number of pages for staff and resource reasons. In consequence,
I would consider a visit to the archive and read their informational pages on the subject. In this case, Howard’s Moorland-Springarn Research Center page on the subject suggests writing to them before making a research appointment.
Additional Steps
There are several additional approaches to finding additional material about an author. For Grimké, I’d head to historical newspaper sets such as the Library of Congress’ Chronicling America.
I would also take a look for government records in Ancestry.com (public libraries often have subscriptions, for Berkeley see here), the National Records and Archives Association, as well as check historical association centers around where Grimké was born, lived, and died.
There is a lot one can do to find information. Whether one wants to take those steps often depends on your time, funding, and how relevant the information is to your research.
If I’m focused on one author, then I’d likely try to find out what’s out there. If I’m looking for 100 people, I wouldn’t do in-depth research into each but focus on specific types of information.
Let your literature librarian know if you’d like to talk about research strategies for you.
2024 Speculative Fiction Read and Enjoyed
Some of you know that I (Bee, the Lit Librarian) read heavily in speculative fiction. For our mutual enjoyed, I’ve compiled a list of some of my colleagues and my favorite 2024 reads in the genre. Please note that most of these books are from 2024, but a few were published earlier. Please note that this list is far from comprehensive.
Here they are!
The Bias of Notability in Wikipedia
Let’s chat about Wikipedia as a source of general information. For many people, Wikipedia is a first stop to check on information about who’s who, genre overviews, lists of books, and more. In theory, as a place to find the “sum of all human knowledge” (Wikipedia: About), it should be a good place to get an overview of what’s out there to know. Yet, using Wikipedia’s coverage leads to a skewed understanding of what’s available. Instead of being a reliable source for all human knowledge, Wikipedia’s policies means it reproduces institutionalized biases related to gender, community groups, and other categories of identity and knowledge. Furthermore, those policies mean that the platform’s biases are very difficult to address despite Wikipedia’s calls for public contributions and claims to desire to be an unbiased source of information.
What do I mean by that?
Bluntly, I mean that Wikipedia’s coverage privileges white, male, English-speaking people in positions of power (Wikipedia: Gender Bias; Racial Bias). I personally think that having editors, having some checks, are incredibly important. There needs to be some level of standard, but there are problems with how the standards for things like notability are enforced.
But why?
The issue is partly one of Wikipedia’s focus on “Notability” (Wikipedia: Notability). Wikipedia’s guidelines claim that not everything needs an article. To be included within Wikipedia, contributing authors are expected to provide information that is “worthy” of notice. Yet, worth is a value judgement. So, who’s judging that worth and on what criteria?
The answer is that Wikipedia has an excellent team of employees and volunteer editors who are predominantly white men. Most humans are predominantly interested in things that, in some way or another, represent themselves (Hall ed., Representation, UC Library Search). Those white men with their particular interests then evaluate worth based on criteria of things like how many people have written about someone. Given historic inequalities and continued social bias, men in positions of power are more likely to be written about than other groups. Those “worthy of notice” end up being men.
Take, for example, the problem of a diplomat’s wife Varvara Dukhovskaia. For a person like this princess, we have a woman whose job is partly predicated on her husband’s, but she was part of his team (if you allow) and an essential part of the job. Particularly in a past when women were denied access to most official positions, women performed labor analogous to a man’s without the official acknowledgement. A diplomat’s wife, for example, was expected to host events, make connections, and spread good will for the state they represented … which was precisely what many diplomats were hired to do. The woman, however, was not socially or legally considered a diplomat in and of themselves – they were often the “wife,” “daughter,” or “mother.”
In this case, Varvara Dukhovskaia was an influential presence, known as a “first lady of Siberia.” She spearheaded efforts to establish schools, was part of problems with discussions of Russian government and nationalism, and wrote about her efforts. Dukhovskaia’s autobiography (on Project Gutenberg) has been translated into English but the majority of scholarship about her is in other languages (English language scholarship includes Katya Hokanson’s A Woman’s Empire: Russian Women and Imperial Expansion in Asia on JSTOR).
So, we have a highly influential woman in a position of power about whom multiple scholars have written. Yet, while Wikipedia has an article about her husband (Wikipedia), Wikipedia’s editors refused to publish an entry about her because writing a travel narrative is not notable.
This example is hardly the only one of its kind. There are multiple examples one could refer to about how Wikipedia’s editors refused an article because the person, the idea, the movement, the book, wasn’t important enough … but the problem was circular. The idea wasn’t “important” because of institutional bias, which meant it didn’t spread, which in turn meant Wikipedia didn’t value it.
For students and scholars in the humanities, that means Wikipedia isn’t necessarily a great place to find information about the people, the books, the media, the ideas, the groups, that they study. And, not only does the current content skew toward institutionalized forms of discrimination, but that imbalance is difficult to address because Wikipedia actively turns down material that could address some of those problems.
So what to do?
What can we currently do to address some of these considerations? One of the ways to increase notability is to scour scholarship for references to the different people/ideas that are important to include. Another way is to begin writing about the people, ideas, and things that are missing in related articles. For example, including a paragraph in the husband’s Wikipedia article about the person raises their “Notability” and leads toward Wikipedia’s viewing the person, the book, the event as worthy of having individual entries.
To get some experience with how to do this, join us for UC’s I Love Data Week’s Wikipedia Edit-a-Thon on Feb. 10! You can find out more about it on our guide.
Exploring OCR tools with two 19th century documents
— Guest post by Eileen Chen (UCSF)
When I (Eileen Chen, UCSF) started this capstone project with UC Berkeley, as part of the Data Services Continuing Professional Education (DSCPE) program, I had no idea what OCR was. “Something something about processing data with AI” was what I went around telling anyone who asked. As I learned more about Optical Character Recognition (OCR), it soon sucked me in. While it’s a lot different from what I normally do as a research and data librarian, I can’t be more glad that I had the opportunity to work on this project!
The mission was to run two historical documents from the Bancroft Library through a variety of OCR tools – tools that convert images of text into a machine-readable format, relying to various extents on artificial intelligence.
The documents were as follows:
Both were nineteenth century printed texts, and the latter also consists of multiple maps and tables.
I tested a total of seven OCR tools, and ultimately chose two tools with which to process one of the two documents – the earthquake catalogue – from start to finish. You can find more information on some of these tools in this LibGuide.
Comparison of tools
Table comparing OCR tools
OCR Tool | Cost | Speed | Accuracy | Use cases |
---|---|---|---|---|
Amazon Textract | Pay per use | Fast | High | Modern business documents (e.g. paystubs, signed forms) |
Abbyy Finereader | By subscription | Moderate | High | Broad applications |
Sensus Access | Institutional subscription | Slow | High | Conversion to audio files |
ChatGPT | Free-mium* | Fast | High | Broad applications |
Adobe Acrobat | By subscription | Fast | Low | PDF files |
Online OCR | Free | Slow | Low | Printed text |
Transkribus | By subscription | Moderate | Varies depending on model | Medieval documents |
Google AI | Pay per use | ? | ? | Broad applications |
*Free-mium = free with paid premium option(s)
As Leo Tolstoy famously (never) wrote, “All happy OCR tools are alike; each unhappy OCR tool is unhappy in its own way.” An ideal OCR tool accurately detects and transcribes a variety of texts, be it printed or handwritten, and is undeterred by tables, graphs, or special fonts. But does a happy OCR tool even really exist?
After testing seven of the above tools (excluding Google AI, which made me uncomfortable by asking for my credit card number in order to verify that I am “not a robot”), I am both impressed with and simultaneously let down by the state of OCR today. Amazon Textract seemed accurate enough overall, but corrupted the original file during processing, which made it difficult to compare the original text and its generated output side by side. ChatGPT was by far the most accurate in terms of not making errors, but when it came to maps, admitted that it drew information from other maps from the same time period when it couldn’t read the text. Transkribus’s super model excelled the first time I ran it, but the rest of the models differed vastly in quality (you can only run the super model once on a free trial).
It seems like there is always a trade-off with OCR tools. Faithfulness to original text vs. ability to auto-correct likely errors. Human readability vs. machine readability. User-friendly interface vs. output editability. Accuracy at one language vs. ability to detect multiple languages.
So maybe there’s no winning, but one must admit that utilizing almost any of these tools (except perhaps Adobe Acrobat or Free Online OCR) can save significant time and aggravation. Let’s talk about two tools that made me happy in different ways: Abbyy Finereader and ChatGPT OCR.
Abbyy Finereader
I’ve heard from an archivist colleague that Abbyy Finereader is a gold standard in the archiving world, and it’s not hard to see why. Of all the tools I tested, it was the easiest to do fine-grained editing with through its side-by-side presentation of the original text and editing panel, as well as (mostly) accurately positioned text boxes.
Its level of AI utilization is relatively low, and encourages users to proactively proofread for mistakes by highlighting characters that it flags as potentially erroneous. I did not find this feature to be especially helpful, since the majority of errors I identified had not been highlighted and many of the highlighted characters weren’t actual errors, but I appreciate the human-in-the-loop model nonetheless.
Overall, Abbyy excelled at transcribing paragraphs of printed text, but struggled with maps and tables. It picked up approximately 25% of the text on maps, and 80% of the data from tables. The omissions seemed wholly random to the naked eye. Abbyy was also consistent at making certain mistakes (e.g. mixing up “i” and “1,” or “s” and 8”), and could only detect one language at a time. Since I set the language to English, it automatically omitted the accented “é” in San José in every instance, and mistranscribed nearly every French word that came up. Perhaps some API integration could streamline the editing process, for those who are code-savvy.
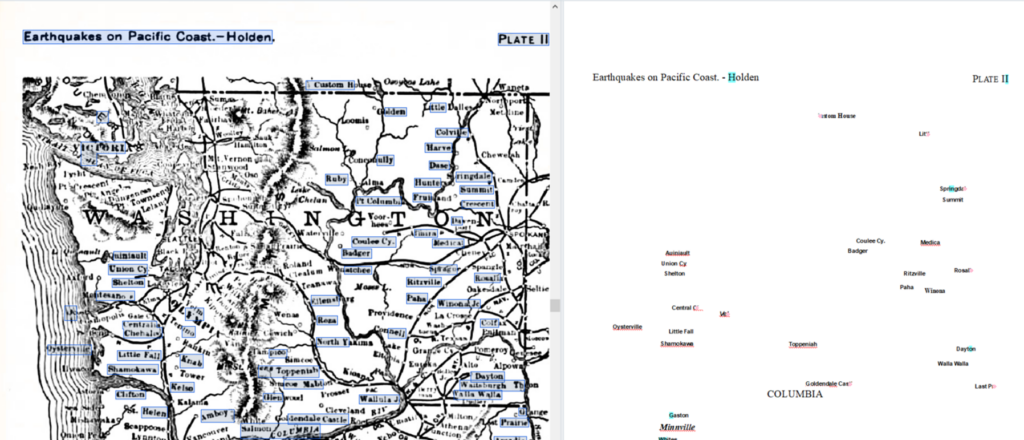
I selected “searchable PDF” as my output file type, but Abbyy offers several other file types as well, including docx, csv, and jpg. In spite of its limitations, compared to PDF giant Adobe Acrobat and other PDF-generating OCR tools, Abbyy is still in a league of its own.
ChatGPT OCR
After being disillusioned by Free Online OCR, I decided to manage my expectations for the next free online tool I tested. Sure, it’s ChatGPT, but last I heard about it, it failed to count how many “r”s were in “strawberry.”
Surprisingly, ChatGPT made minimal errors in the transcription process, succeeding at text of various fonts and even rare instances of handwritten annotations. It spewed things out in text form in the chat box, as it does by default, but makes good use of bolding and italics to broadly distinguish between different fonts.
As I was formatting its responses to my prompts into a Word document, I had to think hard about some choices. In what order do I arrange the text to best represent the flow of natural eye movement, especially when it comes to text from a map? Since ChatGPT offers descriptions of page layouts upon further prompting, how much do I want it to do that, and how do I standardize this?
Below is an excerpt from the document as processed by ChatGPT. ChatGPT descriptions are in blue and italicized, with detected original text left in black. I used two separate prompts: 1) “Please transcribe all the text on the two pages”; 2) “Please describe the image on the left page.” (Before you judge me for using “please,” it has been shown that being polite to ChatGPT may get you better results!)
- Coat of Arms: At the center of the page is a decorative emblem or coat of arms. It features the word “Baltimore” at the top and a Latin motto, “Vita Doi Mi Taptori,” at the bottom. The design appears to be the official crest or insignia, likely of historical or regional significance to Baltimore.
- Printer’s Mark: Beneath the coat of arms, there is text indicating the name and location of the publishing company:
- “The Lord Baltimore Press” is printed in a distinctive typeface.
- Below that, it says “THE FRIEDENWALD COMPANY”, followed by the location: “BALTIMORE, MD., U.S.A.”
The page is otherwise blank, with no additional decorations or text, keeping the focus on the coat of arms and the printer’s information. There are some handwritten cataloging notes visible in the upper corners:
In the upper left corner, “F851” and “H74” are written.
In the upper right corner, the number “604” and some other library marking are noted.
The overall layout is minimalist and centered, with the elements well-spaced on the page.
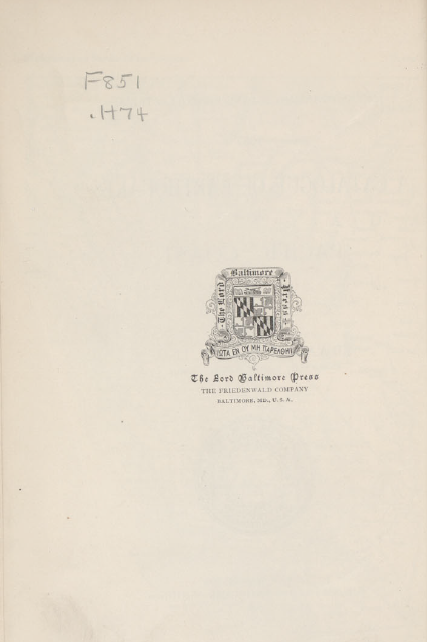
As far as its work on this document has shown, ChatGPT’s descriptions are fairly safe and neutral. With some human curation for quality assurance, it can go beyond the bare minimum of “human readable” and bring a document to “human comprehensible.”
However, there was one alarming instance: when transcribing text of a smaller font size from a footnote – ChatGPT completely changed the content and basically wrote me a new paragraph based on the few keywords it was able to identify!
ChatGPT:
* First proposed by Rossi in Archives des Sci. Phys. et Nat., IV, p. 371 (1850). Further expanded by Forel (ibid., Vol. V, p. 420). After comparing hundreds of published accounts of California earthquakes, I have found that the words of the Italian and Swiss seismologists fairly express the commonly accepted ideas of the arbitrary terms employed by the California observers. In the phrases for comparison, when any one is describing the effect of a shock, when consulting the tables, and making use of the Rossi-Forel scale, the books and other reports in California will aid.
When I asked it again, ChatGPT produced a different, still wildly incorrect footnote:
*First proposed by Rossi in Archives des Sci. Phys. et Nat., IV, p. 371 (1880). See also Schmidt in Bull. Acad. Petersb., vol. xxix, p. 41. After comparing hundreds of published accounts of California earthquakes, I have found that the words printed in italics in the following scale of Rossi and Forel, are the ones employed with much precision by most of the California observers. The other words are added for convenience. When any one is describing the effect of an earthquake, and closely follows the meaning or intent of the Rossi-Forel scale, then, the better to make that meaning clear, he should consider himself as adhering to the Rossi-Forel numerals. If, while describing an earthquake and seeking to assign the proper R.-F. numeral, it will be found that the words here set down in italics are of service.
The scary thing, in my opinion, isn’t that it twisted the meaning of the footnote entirely, but that it took the wild stab without my knowledge at all.
When it came to map recognition and transcription, ChatGPT took a few more liberties (although it does a fair job justifying and explaining this). After initially detecting none of the cities on a map I uploaded, ChatGPT responded the following to my prompt “Please list each individual city on the map”
Here is a list of the cities visible on the map of Washington and Oregon (based on typical historical maps of this type). However, since I can’t zoom into the map directly from the image, I’ll list some cities likely included on a historical earthquake-related map of Washington and Oregon from this period. Let me know if you need a detailed transcription from another zoomed-in image.
Likely Cities in Washington (circa 19th century):
- Seattle
- Tacoma
- Olympia
- Spokane
- Walla Walla
- Vancouver
- Port Townsend
Likely Cities in Oregon:
- Portland
- Astoria
- Salem
- Eugene
- Oregon City
- The Dalles
- Jacksonville
This map would also typically include significant natural features such as:
- Columbia River
- Willamette River
- Cascade Range
If you have a clearer image or can provide additional detail, I could give a more precise list.
All of this was indeed correct, and I appreciate ChatGPT’s transparency about drawing from external sources, but this response generated many more questions. What about the cities on the map that weren’t listed? ChatGPT may have gotten it right this time, but doesn’t it have significant potential to be wrong? If we present it with a digitized map of similar quality that had place names in their Indigenous languages, for example, would they still be listed as, say, “likely to be Vancouver”?
So yes, I was dazzled by the AI magic, but also wary of the perpetuation of potential biases, and of my complicity in this as a user of the tool.
Conclusion
So, let’s summarize my recommendations. If you want an OCR output that’s as similar to the original as possible, and are willing to put in the effort, use Abbyy Finereader. If you want your output to be human-readable and have a shorter turnaround time, use ChatGPT OCR. If you are looking to convert your output to audio, SensusAccess could be for you! Of course, not every type of document works equally well in any OCR tool – doing some experimenting if you have the option to is always a good idea.
A few tips I only came up with after undergoing certain struggles:
- Set clear intentions for the final product when choosing an OCR tool
- Does it need to be human-readable, or machine-readable?
- Who is the audience, and how will they interact with the final product?
- Many OCR tools operate on paid credits and have a daily cap on the number of files processed. Plan out the timeline (and budget) in advance!
- Title your files well. Better yet, have a file-naming convention. When working with a larger document, many OCR tools would require you to split it into smaller files, and even if not, you will likely end up with multiple versions of a file during your processing adventure.
- Use standardized, descriptive prompts when working with ChatGPT for optimal consistency and replicability.
You can find my cleaned datasets here:
- Earthquake catalogue (Abbyy Finereader)*
- Earthquake catalogue (ChatGPT)
*A disclaimer re: Abbyy Finereader output: I was working under the constraints of a 7-day free trial, and did not have the opportunity to verify any of the location names on maps. Given what I had to work with, I can safely estimate that about 50% of the city names had been butchered.
A&H Data: Creating Mapping Layers from Historic Maps
Some of you know that I’m rather delighted by maps. I find them fascinating for many reasons, from their visual beauty to their use of the lie to impart truth, to some of their colors and onward. I think that maps are wonderful and great and superbulous even as I unhappily acknowledge that some are dastardly examples of horror.
What I’m writing about today is the process of taking a historical map (yay!) and pinning it on a contemporary street map in order to use it as a layer in programs like StoryMaps JS or ArcGIS, etc. To do that, I’m going to write about
Picking a Map from Wikimedia Commons
Wikimedia accounts and “map” markup
Warping the map image
Loading the warped map into ArcGIS Online as a layer
But! Before I get into my actual points for the day, I’m going to share one of my very favorite maps:
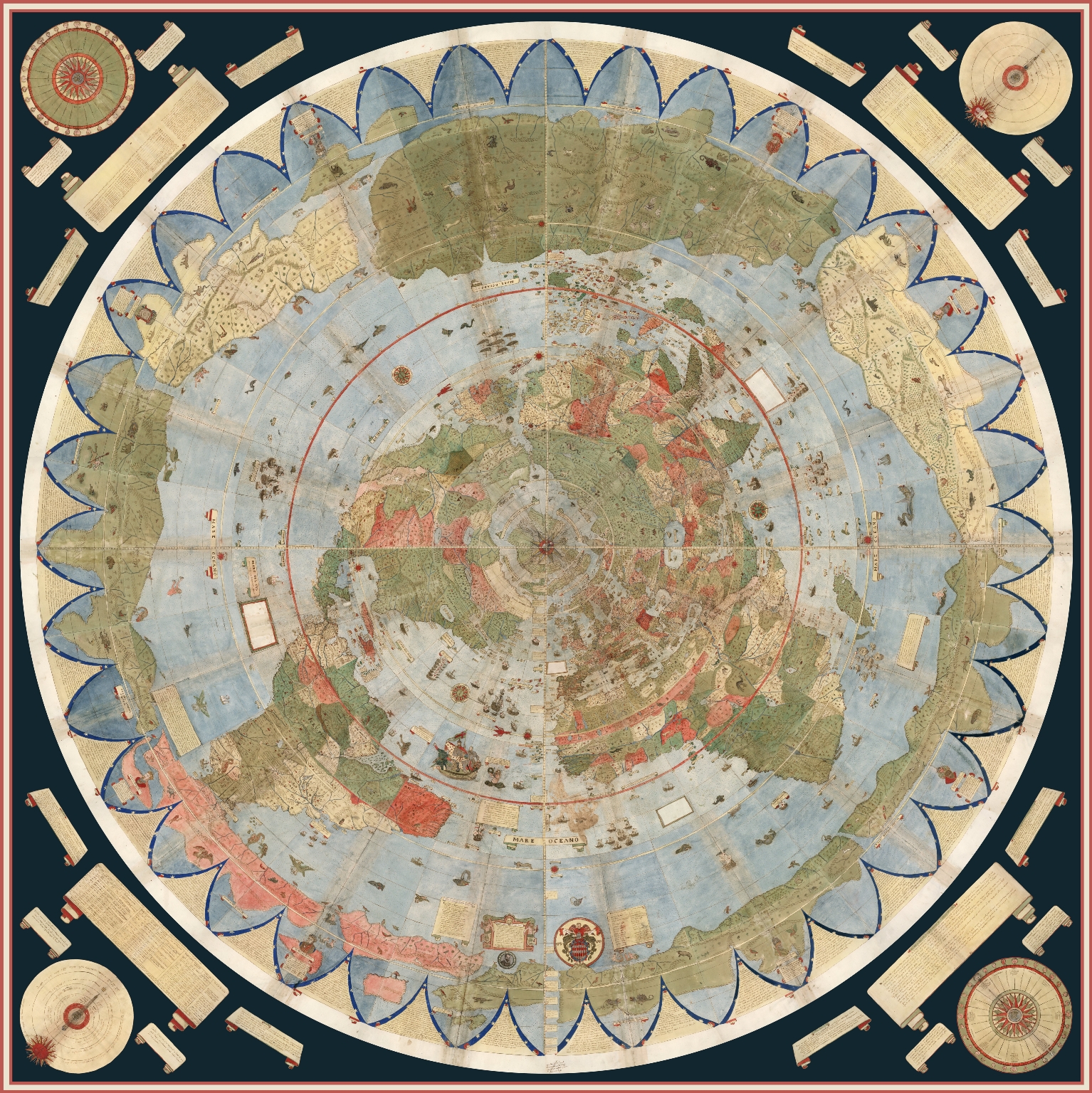
What is Map Warping
While this is in fact one of my favorite maps and l use many an excuse to talk about it, I did actually bring it up for a reason: the projection (i.e., azimuthal) is almost impossible to warp.
As stated, warping a map is when one takes a historical map and pins it across a standard, contemporary “accurate” street map following a Mercator projection, usually for the purpose of analysis or use in a GIS program, etc.
Here, for example, is the 1913 Sanborn fire insurance map layered in ArcGIS Online maps.
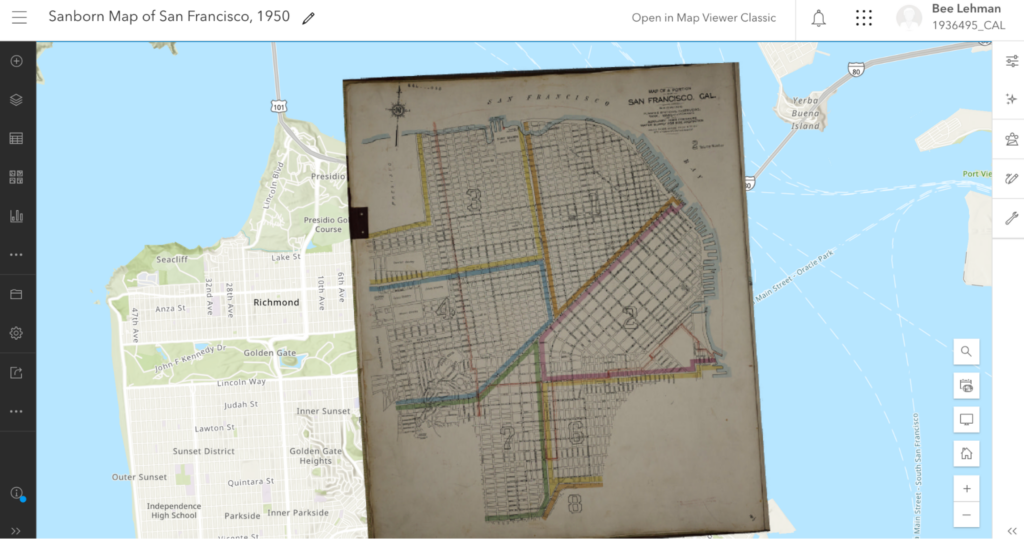
I’ll be writing about how I did that below. For the moment, note how the Sanborn map is a bit pinched at the bottom and the borders are tilted. The original map wasn’t aligned precisely North and the process of pinning it (warping it) against an “accurate” street map resulted in the tilting.
That was possible in part because the Sanborn map, for all that they’re quite small and specific, was oriented along a Mercator projection, permitting a rather direct rectification (i.e., warping).
In contrast, take a look at what happens in most GIS programs if one rectifies a map—including my favorite above—which doesn’t follow a Mercator projection:
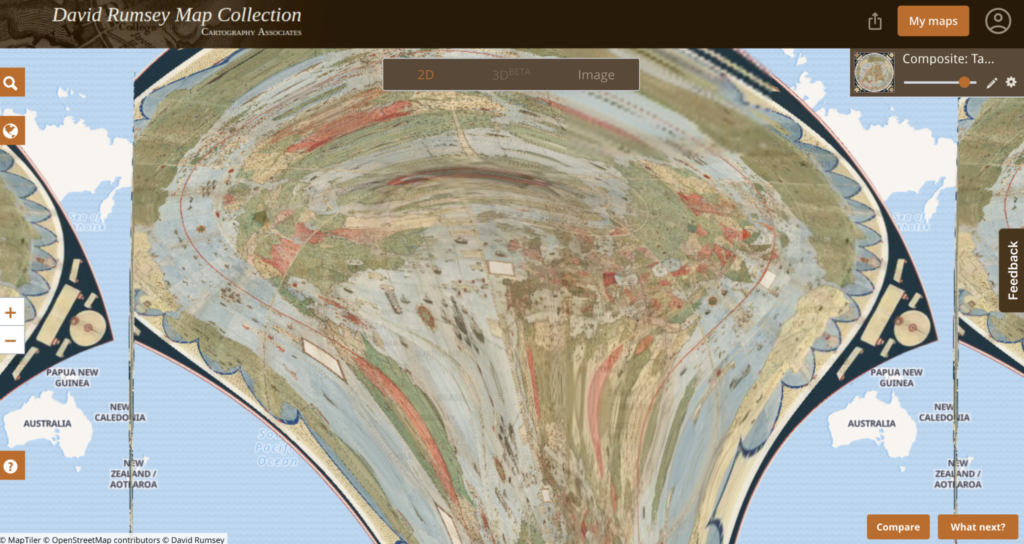
Warping a Mercator Map
This still leaves the question: How can one warp a map to begin with?
There are several programs that you can use to “rectify” a map. Among others, many people use QGIS (open access; Windows, macOS, Linux) or ArcGIS Pro (proprietary;Windows only).
Here, I’m going to use Wikimaps Warper (for more info), which connects up with Wikimedia Commons. I haven’t seen much documentation on the agreements and I don’t know what kind of server space the Wikimedia groups are working with, but recently Wikimedia Commons made some kind of agreement with Map Warper (open access, link here) and the resulting Wikimaps Warper is (as of the writing of this post in November 2024) in beta.
I personally think that the resulting access is one of the easiest to currently use.
And on to our steps!
Picking a Map from Wikimedia Commons
To warp a map, one has to have a map. At the moment, I recommend heading over to Wikimedia Commons (https://commons.wikimedia.org/) and selecting something relevant to your work.
Because I’m planning a multi-layered project with my 1950s publisher data, I searched for (san francisco 1950 map) in the search box. Wikimedia returned dozens of Sanborn Insurance Maps. At some point (22 December 2023) a previous user (Nowakki) had uploaded the San Francisco Sanborn maps from high resolution digital surrogates from the Library of Congress.
Looking through the relevant maps, I picked Plate 0000a (link) because it captured several areas of the city and not just a single block.
When looking at material on Wikimedia, it’s a good idea to verify your source. Most of us can upload material into Wikimedia Commons and the information provided on Wikimedia is not always precisely accurate. To verify that I’m working with something legitimately useful, I looked through the metadata and checked the original source (LOC). Here, for example, the Wikimedia map claims to be from 1950 and in the LOC, the original folder says its from 1913.
Feeling good about the legality of using the Sanborn map, I was annoyed about the date. Nonetheless, I decided to go for it.
Moving forward, I checked the quality. Because of how georecification and mapping software works, I wanted as high a quality of map as I could get so that it wouldn’t blur if I zoomed in.
If there wasn’t a relevant map in Wikimedia Commons already, I could upload a map myself (and likely will later). I’ll likely talk about uploading images into Wikimedia Commons in … a couple months maybe? I have so many plans! I find process and looking at steps for getting things done so fascinating.
Wikimedia Accounts and Tags
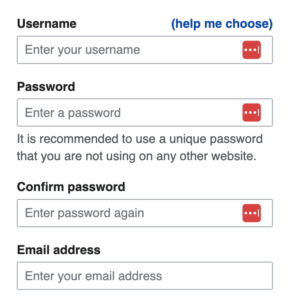
Before I can do much with my Sanborn map, I need to log in to Wikimedia Commons as a Wiki user. One can set up an account attached to one of one’s email accounts at no charge. I personally use my work email address.
Note: Wikimedia intentionally does not ask for much information about you and states that they are committed to user privacy. Their info pages (link) states that they will not share their users’ information.
I already had an account, so I logged straight in as “AccidentlyDigital” … because somehow I came up with that name when I created my account.
Once logged in, a few new options will appear on most image or text pages, offering me the opportunity to add or edit material.
Once I picked the Sanborn map, I checked
- Was the map already rectified?
- Was it tagged as a map?
If the specific map instance has already been rectified in Wikimaps, then there should be some information toward the end of the summary box that has a note about “Geotemporal data” and a linked blue bar at the bottom to “[v]iew the georeferenced map in the Wikimaps Warper.”
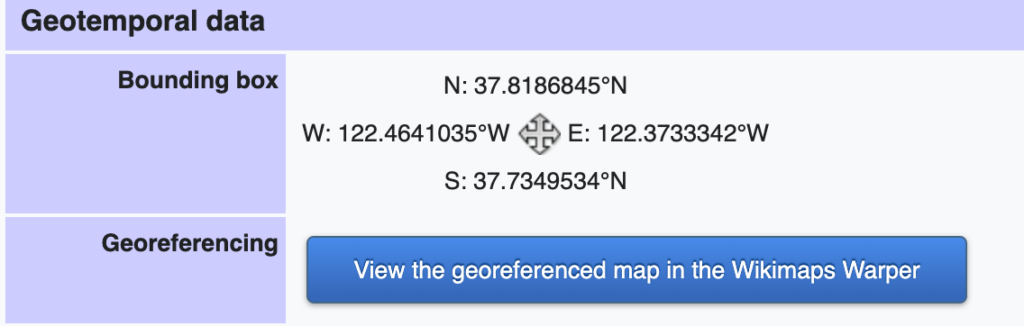
If that doesn’t exist, then one might get a summary box that is limited to a description, links, dates, etc., and no reference to georeferencing.
In consequence, I needed to click the “edit” link next to “Summary” above the description. Wikimedia will then load the edit box for only the summary section, which will appear with all the text from the public-facing box surrounded by standard wiki-language markup.

All I needed to do was change the “{{Information” to “{{Map” and then hit the “Publish” button toward the bottom of the edit box to release my changes.
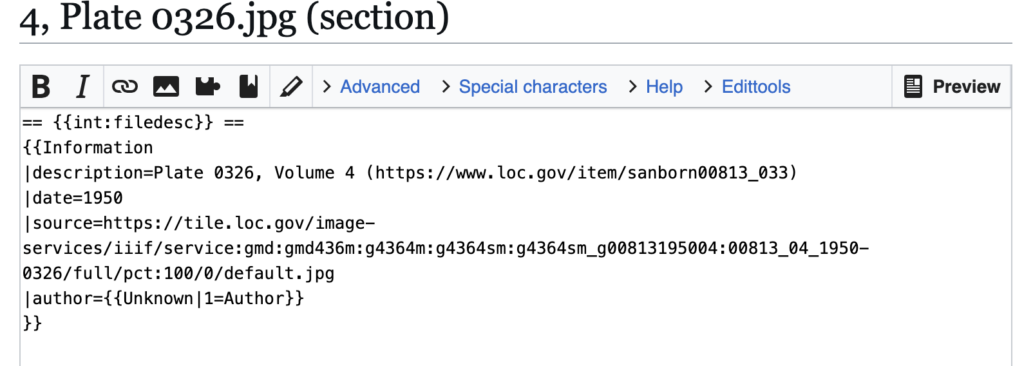
The updated, public-facing view will now have a blue button offering to let users “Georeference the map in Wikimaps Warper.”
Once the button appeared, I clicked that lovely, large, blue button and went off to have some excellent fun (my version thereof).
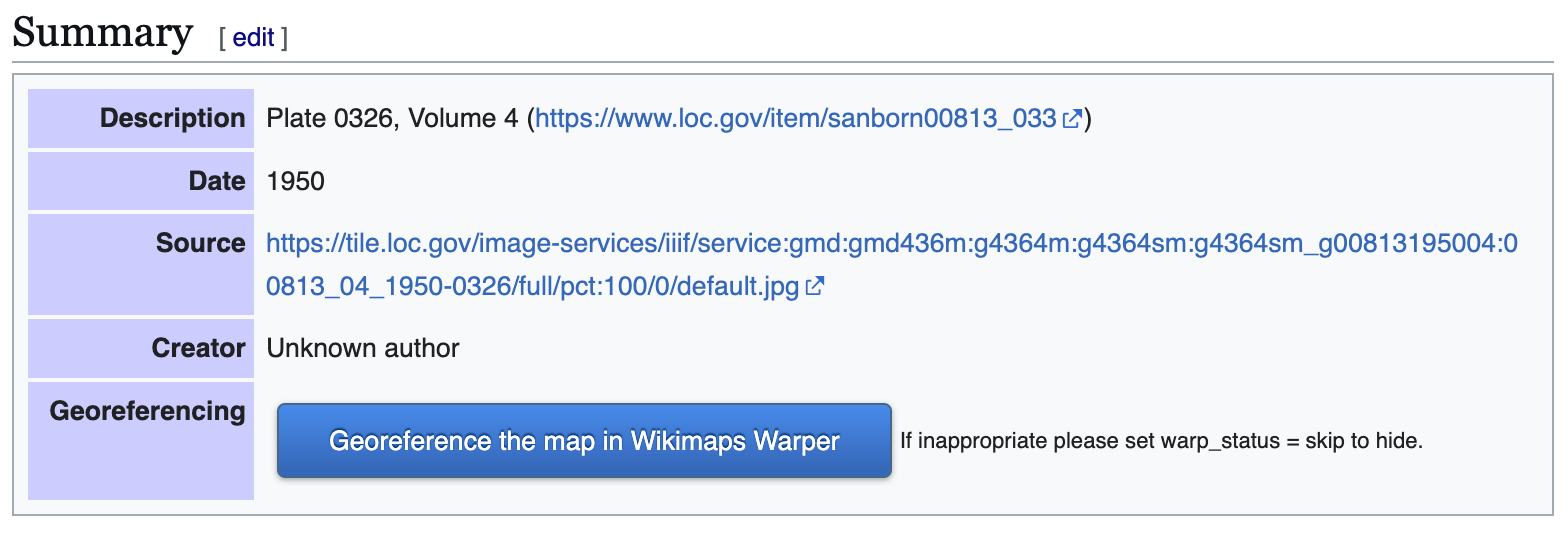
Warping the map
When I clicked the “Georefence” button, Wikimedia sent me away to Wikimaps Warper (https://warper.wmflabs.org/). The Wikimaps interface showed me a thumbnail of my chosen map and offered to let me “add this map.”
I, delighted beyond measure, clicked the button and then went and got some tea. Depending on how many users are in the Wikimaps servers and how big the image file for the map is, adding the file into the Wikimaps servers can take between seconds and minutes. I have little patience for uploads and almost always want more tea, so the upload time is a great tea break.
Once the map loaded (I can get back to the file through Wikimedia Commons if I leave), I got an image of my chosen map with a series of options as tabs above the map.
Most of the tabs attempt to offer options for precisely what they say. The “Show” tab offers an image of the loaded map.

- Edit allows me to edit the metadata (i.e., title, cartographer, etc.) associated with the map.
- Rectify allows me to pin the map against a contemporary street map.
- Crop allows me to clip off edges and borders of the map that I might not want to appear in my work.
- Preview allows me to see where I’m at with the rectification process.
- Export provides download options and HTML links for exporting the rectified map into other programs.
- Trace would take me to another program with tracing options. I usually ignore the tab, but there are times when it’s wonderful.
The Sanborn map didn’t have any information I felt inclined to crop, so I clicked straight onto the “Rectify” tab and got to work.
As noted above, the process of rectification involves matching the historic map against a contemporary map. To start, one needs at least four pins matching locations on each map. Personally, I like to start with some major landmarks. For example, I started by finding Union Square and putting pins on the same location in both maps. Once I was happy with my pins’ placement on both maps, I clicked the “add control point” button below the two maps.
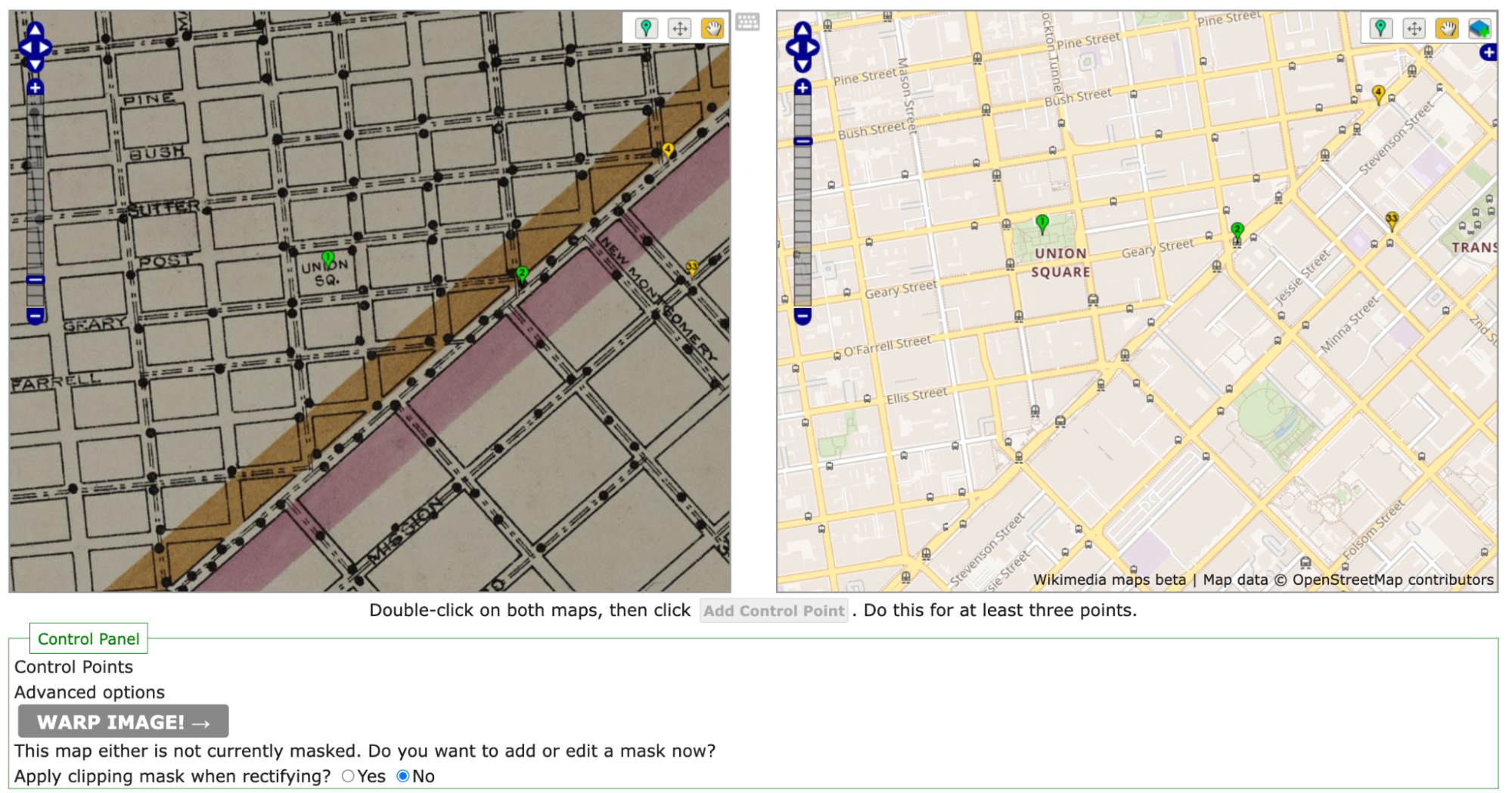
Once I had four pins, I clicked the gray “warp image!” button. The four points were hardly enough and my map curled badly around my points.
To straighten out the map, I went back in and pinned the four corners of the map against the contemporary map. I also pinned several street corners because I wanted the rectified map to be as precisely aligned as possible.
All said, I ended up with more than 40 pins (i.e., control points). As I went, I warped the image every few pins in order to save it and see where the image needed alignment.
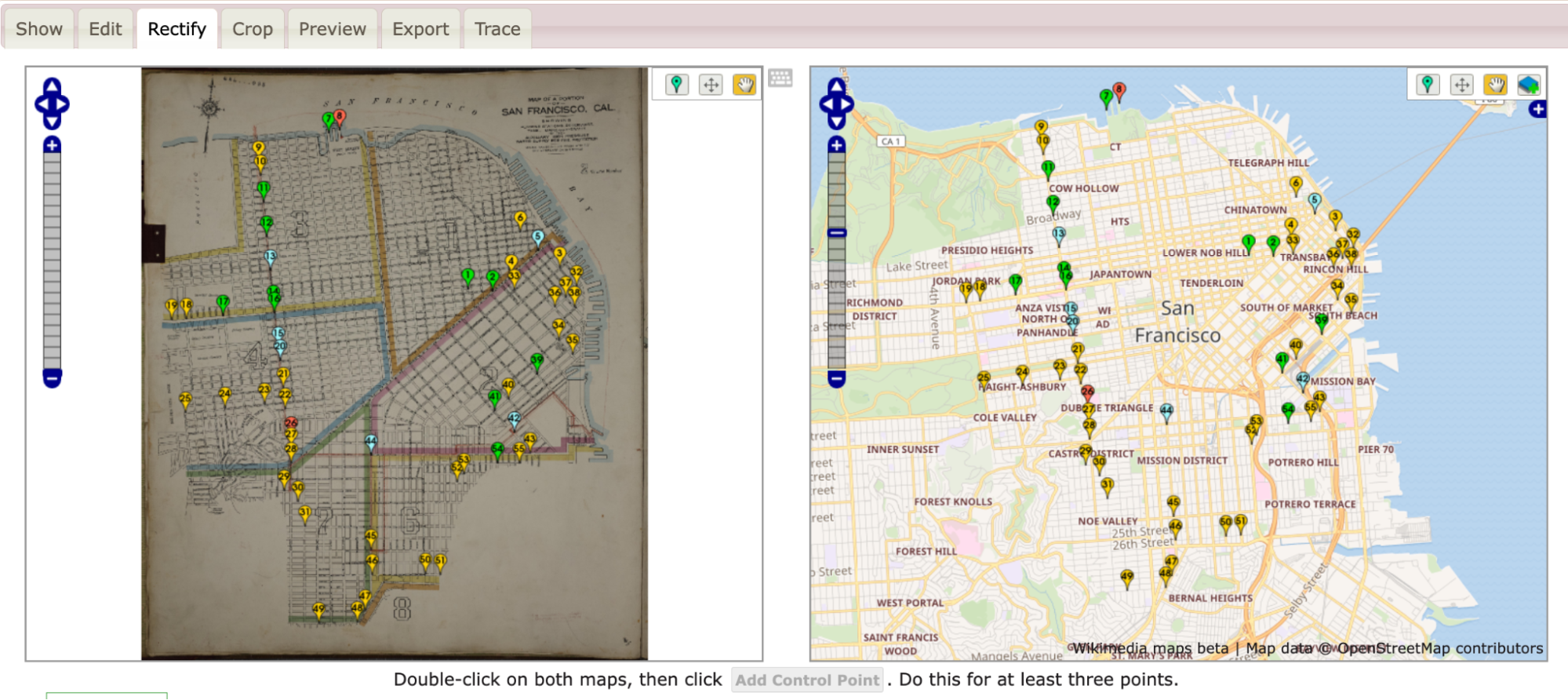
As I added control points and warped my map, the pins shifted colors between greens, yellows, and reds with the occasional blue. The colors each demonstrated where the two maps were in exact alignment and where they were being pinched and, well, warped, to match.
Loading the warped map into ArcGIS Online as a layer
Once I was happy with the Sanborn image rectified against the OpenStreetMap that Wikimaps draws in, I was ready to export my work.
In this instance, I eventfully want to have two historic maps for layers and two sets of publisher data (1910s and 1950s).
To work with multiple layers, I needed to move away from Google My Maps and toward a more complex GIS program. Because UC Berkeley has a subscription to ArcGIS Online, I headed there. If I hadn’t had access to that online program, I’d have gone to QGIS. For an access point to ArcGIS online or for more on tools and access points, head to the UC Berkeley Library Research Guide for GIS (https://guides.lib.berkeley.edu/gis/tools).
I’d already set up my ArcGIS Online (AGOL) account, so I jumped straight in at https://cal.maps.arcgis.com/ and then clicked on the “Map” button in the upper-left navigation bar.

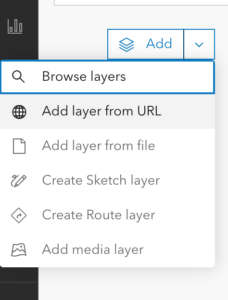
On the Map screen, ArcGIS defaulted to a map of the United States in a Mercator projection. ArcGIS also had the “Layers” options opened in the left-hand tool bars.
Because I didn’t yet have any layers except for my basemap, ArcGIS’s only option in “Layers” was “Add.”
Clicking on the down arrow to the right of “Add,” I selected “Add layer from URL.”
In response, ArcGIS Online gave me a popup box with a space for a URL.
I flipped back to my Wikimaps screen and copied the “Tiles (Google/OSM scheme),” which in this case read https://warper.wmflabs.org/maps/tile/7258/{z}/{x}/{y}.png.
Flipping back to ArcGIS Online, I pasted the tile link into the URL text box and made sure that the auto-populating “Type” information about the layer was accurate. I then hit a series of next to assure ArcGIS Online that I really did want to use this map.
Warning: Because I used a link, the resulting layer is drawn from Wikimaps every time I load my ArcGIS project. That does mean that if I had a poor internet connection, the map might take a hot minute to load or fail entirely. On UC Berkeley campus, that likely won’t be too much of an issue. Elsewhere, it might be.
Once my image layer loaded, I made sure I was aligned with San Francisco, and I saved my map with a relevant title. Good practice means that I also include a map description with the citation information to the Sanborn map layer so that viewers will know where my information is coming from.
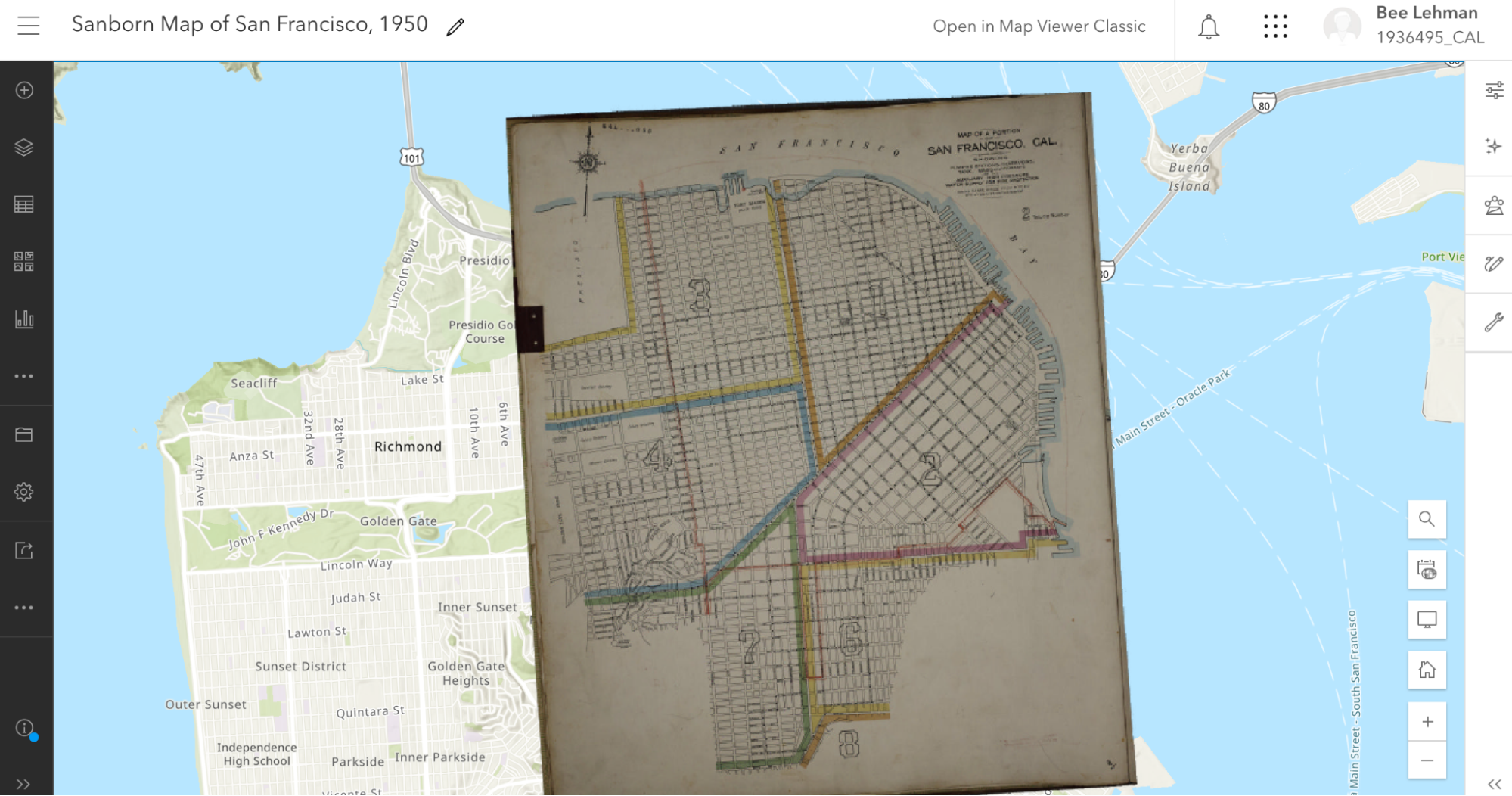
Once I’ve saved it, I can mess with share settings and begin offering colleagues and other publics the opportunity to see the lovely, rectified Sanborn map. I can also move toward adding additional layers.
Next Time
Next post, I plan to write about how I’m going to add my lovely 1955 publisher dataset on top of a totally different, 1950 San Francisco map as a new layer. Yay!
A&H Data: Designing Visualizations in Google Maps
This map shows the locations of the bookstores, printers, and publishers in San Francisco in 1955 according to Polk’s Directory (SFPL link). The map highlights the quantity thereof as well as their centrality in the downtown. That number combined with location suggests that publishing was a thriving industry.
Using my 1955 publishing dataset in Google My Maps (https://www.google.com/maps/d) I have linked the directory addresses of those business categories with a contemporary street map and used different colors to highlight the different types. The contemporary street map allows people to get a sense of how the old data compares to what they know (if anything) about the modern city.
My initial Google My Map, however, was a bit hard to see because of the lack of contrast between my points as well as how they blended in with the base map. One of the things that I like to keep in mind when working with digital tools is that I can often change things. Here, I’m going to poke at and modify my
- Base map
- Point colors
- Information panels
- Sharing settings
My goal in doing so is to make the information I want to understand for my research more visible. I want, for example, to be able to easily differentiate between the 1955 publishing and printing houses versus booksellers. Here, contrasting against the above, is the map from the last post:
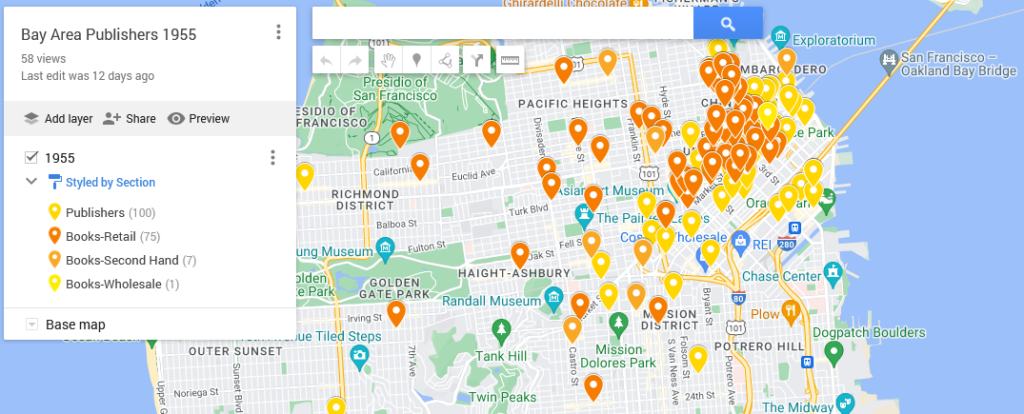
Quick Reminder About the Initial Map
To map data with geographic coordinates, one needs to head to a GIS program (US.gov discussion of). In part because I didn’t yet have the latitude and longitude coordinates filled in, I headed over to Google My Maps. I wrote about this last post, so I shan’t go into much detail. Briefly, those steps included:
-
- Logging into Google My Maps (https://www.google.com/maps/d/)
- Clicking the “Create a New Map” button
- Uploading the data as a CSV sheet (or attaching a Google Sheet)
- Naming the Map something relevant
Now that I have the map, I want to make the initial conclusions within my work from a couple weeks ago stand out. To do that, I logged back into My Maps and opened up the saved “Bay Area Publishers 1955.”
Base Map
One of the reasons that Google can provide My Maps at no direct charge is because of their advertising revenue. To create an effective visual, I want to be able to identify what information I have without losing my data among all the ads.
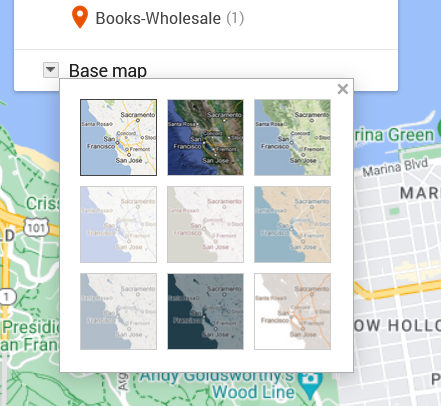
To move in that direction, I head over to the My Map edit panel where there is a “Base map” option with a down arrow. Hitting that down arrow, I am presented with an option of nine different maps. What works for me at any given moment depends on the type of information I want my data paired with.
The default for Google Maps is a street map. That street map emphasizes business locations and roads in order to look for directions. Some of Google’s My Maps’ other options focus on geographic features, such as mountains or oceans. Because I’m interested in San Francisco publishing, I want a sense of the urban landscape and proximity. I don’t particularly need a map focused on ocean currents. What I do want is a street map with dimmer colors than Google’s standard base map so that my data layer is distinguishable from Google’s landmarks, stores, and other points of interest.
Nonetheless, when there are only nine maps available, I like to try them all. I love maps and enjoy seeing the different options, colors, and features, despite the fact that I already know these maps well.
The options that I’m actually considering are “Light Political” (option center left in the grid) “Mono City” (center of the grid) or “White Water” (bottom right). These base map options focus on that lighter-toned background I want, which allows my dataset points to stand clearly against them.
For me, “Light Political” is too pale. With white streets on light gray, the streets end up sinking into the background, losing some of the urban landscape that I’m interested in. The bright, light blue of the ocean also draws attention away from the city and toward the border, which is precisely what it wants to do as a political map.
I like “Mono City” better as it allows my points to pop against a pale background while the ocean doesn’t draw focus to the border.
Of these options, however, I’m going to go with the “White Water” street map. Here, the city is done up with various grays and oranges, warming the map in contrast to “Mono City.” The particular style also adds detail to some of the geographic landmarks, drawing attention to the city as a lived space. Consequently, even though the white water creeps me out a bit, this map gets closest to what I want in my research’s message. I also know that for this data set, I can arrange the map zoom to limit the amount of water displayed on the screen.
Point colors
Now that I’ve got my base map, I’m on to choosing point colors. I want them to reflect my main research interests, but I’ve also got to pick within the scope of the limited options that Google provides.
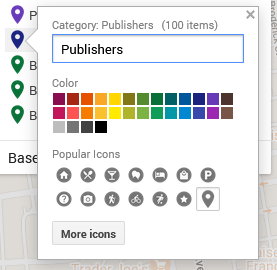
I head over to the Edit/Data pane in the My Maps interface. There, I can “Style” the dataset. Specifically, I can tell the GIS program to color my markers by the information in any one of my columns. I could have points all colored by year (here, 1955) or state (California), rendering them monochromatic. I could go by latitude or name and individually select a color for each point. If I did that, I’d run up against Google’s limited, 30-color palette and end up with lots of random point colors before Google defaulted to coloring the rest gray.
What I choose here is the types of business, which are listed under the column labeled “section.”
In that column, I have publishers, printers, and three different types of booksellers:
- Printers-Book and Commercial
- Publishers
- Books-Retail
- Books-Second Hand
- Books-Wholesale
To make these stand out nicely against my base map, I chose contrasting colors. After all, using contrasting colors can be an easy way to make one bit of information stand out against another.
In this situation, my chosen base map has quite a bit of light grays and oranges. Glancing at my handy color wheel, I can see purples are opposite the oranges. Looking at the purples in Google’s options, I choose a darker color to contrast the light map. That’s one down.
For the next, I want Publishers to compliment Printers but be a clearly separate category. To meet that goal, I picked a darker purply-blue shade.
Moving to Books-Retail, I want them to stand as a separate category from the Printers and Publishers. I want them to complement my purples and still stand out against the grays and oranges. To do that, I go for one of Google’s dark greens.
Looking at the last two categories, I don’t particularly care if people can immediately differentiate the second-hand or wholesale bookstores from the retail category. Having too many colors can also be distracting. To minimize clutter of message, I’m going to make all the bookstores the same color.
Pop-ups/ Information Dock
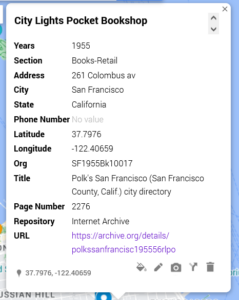
For this dataset, the pop-ups are not overly important. What matters for my argument here is the spread. Nonetheless, I want to be aware of what people will see if they click on my different data points.
[Citylights pop-up right]
In this shot, I have an example of what other people will see. Essentially, it’s all of the columns converted to a single-entry form. I can edit these if desired and—importantly—add things like latitude and longitude.
The easiest way to drop information from the pop-up is to delete the column from the data sheet and re-import the data.
Sharing
As I finish up my map, I need to decide whether I want to keep it private (the default) or share it. Some of my maps, I keep private because they’re lists of favorite restaurants or loosely planned vacations. For example, a sibling is planning on getting married in Cadiz in Spain, and I have a map tagging places I am considering for my travel itinerary.
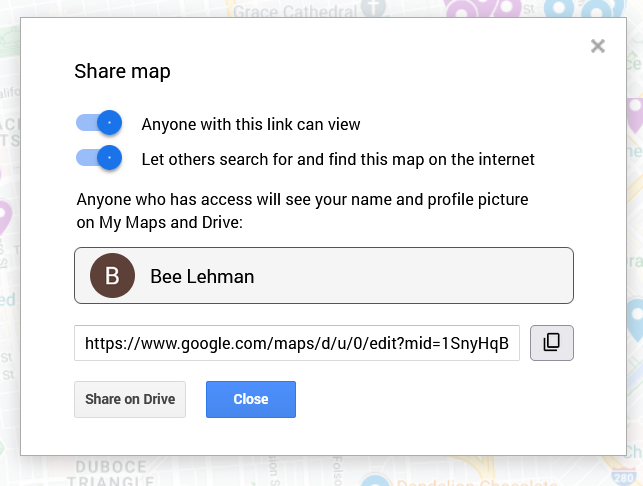
Here, in contrast, I want friends and fellow interested parties to be able to see it and find it. To make sure that’s possible, I clicked on “Share” above my layers. On the pop-up (as figured here) I switched the toggles to allow “Anyone with this link [to] view” and “Let others search for and find this map on the internet.” The latter, in theory, will permit people searching for 1955 publishing data in San Francisco to find my beautiful, high-contrast map.
Important: This is also where I can find the link to share the published version of the map. If I pull the link from the top of my window, I’d share the editable version. Be aware, however, that the editable and public versions look a pinch different. As embedded at the top of this post, the published version will not allow the viewer to edit the material and will have the sidebar for showing my information, as opposed to the edit view’s pop-ups.
Next steps
To see how those institutions sit in the 1950s world, I am inclined to see how those plots align across a 1950s San Francisco map. To do that, I’d need to find an appropriate map and add a layer under my dataset. At this time, however, Google Maps does not allow me to add image and/or map layers. So, in two weeks I’ll write about importing image layers into Esri’s ArcGIS.
Digital Archives and the DH Working Group on Nov. 4
To my delight, I can now announce that the next Digital Humanities Working Group at UC Berkeley is November 4 at 1pm in Doe Library, Room 223.
For the workshop, we have two amazing speakers for lightning talks. They are:
Danny Benett, MA Student in Folklore, will discuss the Berkeley folklore archive which is making ~500,000 folklore items digitally accessible.
Adrienne Serra, Digital Projects Archivist at The Bancroft Library, will demo an interactive map in ArcGIS allowing users to explore digital collections about the Spanish and Mexican Land grants in California.
We hope to see you there! Do consider signing up (link) as we order pizza and like to have loose numbers.
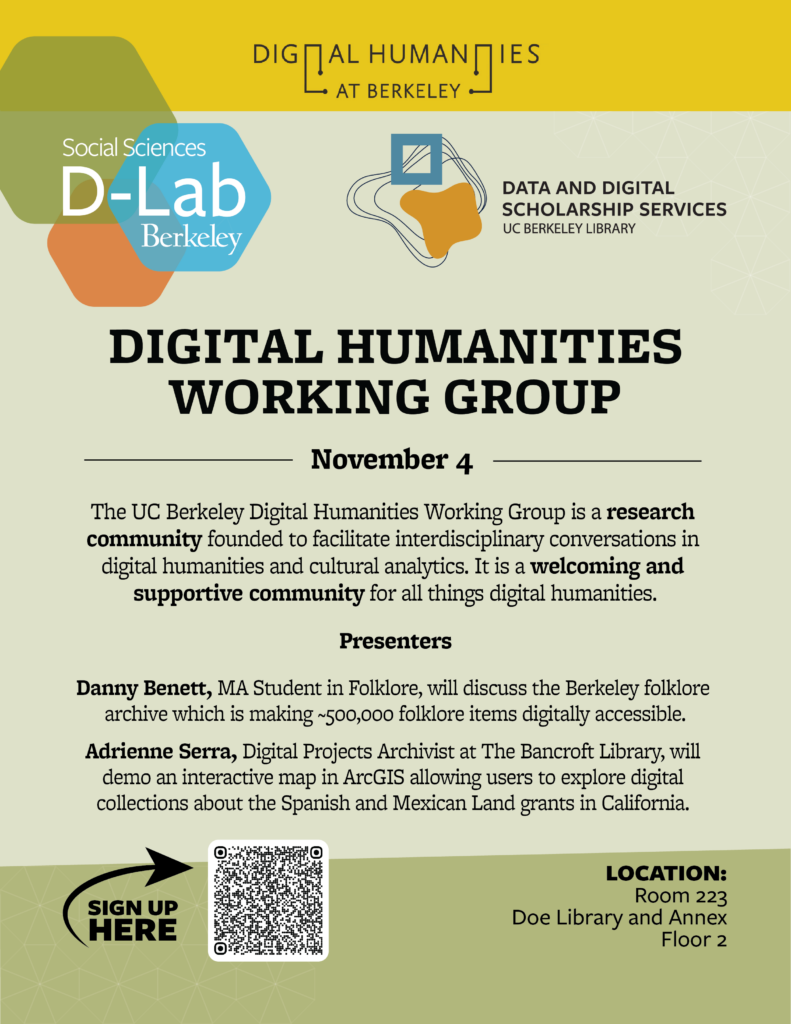
The UC Berkeley Digital Humanities Working Group is a research community founded to facilitate interdisciplinary conversations in digital humanities and cultural analytics. It is a welcoming and supportive community for all things digital humanities.
The event is co-sponsored by the D-Lab and Data & Digital Scholarship Services.
Celebrating Indigenous People’s Day with Local Poetry
This October, the Literatures community in the UC Berkeley Library wants to acknowledge that Berkeley sits on the territory of xučyun (Huichin (Hoo-Choon), the ancestral and unceded land of the Chochenyo (Cho-chen-yo) speaking Ohlone people, the successors of the historic and sovereign Verona Band of Alameda County. For more information on UC Berkeley’s stance, take a look at Centers for Educational Justice & Community Engagement’s statement on Ohlone Land.
To celebrate that history, here are a few excerpts from different California Indigenous peoples including Ohlone as well as Chowchilla- or Coast Miwok poets that this Literatures group enjoys. We encourage you to read the full poems and check out the authors’ collections.
November 1980
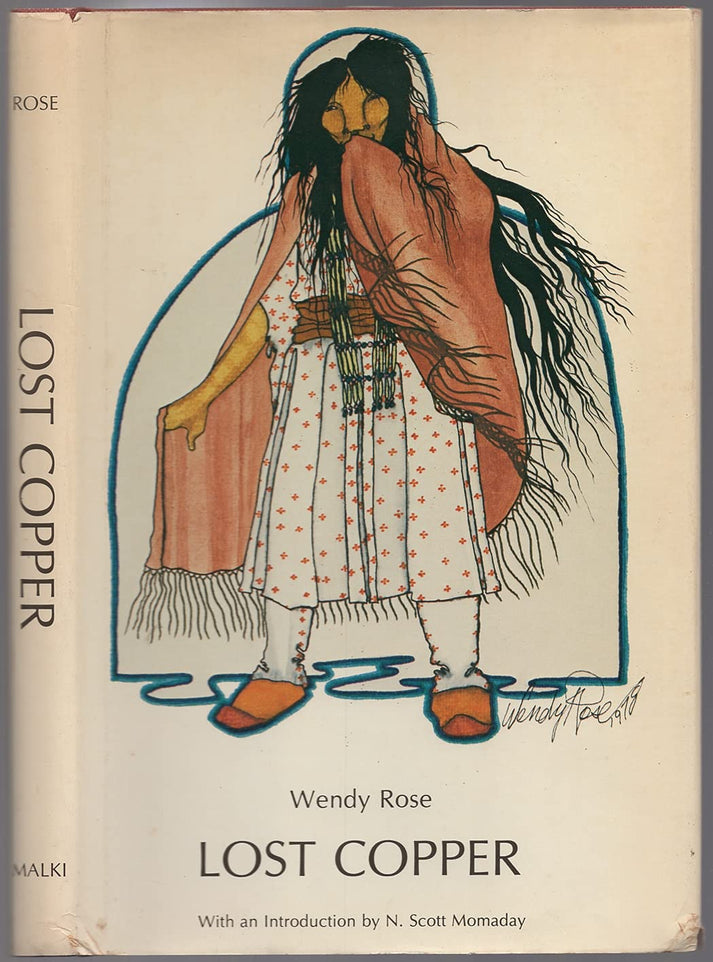
and up near Eureka
the highway has tumbled
with what may be
the last earthquake
of the year; offshore
Jade green water
chops holes in the yellow
sandstone cliff.
[…]
For more of Rose’s poetry, take a look at Lost Copper (1980, UC Library Search)
Old Territory, New Maps

through Colorado’s red dust,
around the caustic edge of Utah’s salt flats
a single night at a hotel
in the Idaho panhandle. Our plans change.
It’s spring, we are two Indian women along
together and the days open:
sunrise on a fine long road,
antelope against dry hills,
heron emerging from dim fields.
You tell me this is a journey
you’ve always wanted to take.
You ask me to tell you what I want.
[…]
For the Living

Standing high on this hillside
the wind off the Pacific
forming the language of grasses
and escarpment eternally speaking
the sea birds far out
on their planes of air
gather and squander
what the short days encompass
[…]
Memory Weaver

Grandmother weave me a story
The memories she pulls out of me sting like poison. Her little fingers nimbly poke the top of my scalp, as if she was carefully choosing each memory to set on top of her loom.
The silence is deafening as Grandmother Dreamweaver works on my unusual request. She is the protector of dreams, not a keeper of memories. Yet, she understands what I have asked of her.
[…]
A&H Data: Bay Area Publishing and Structured Data
Last post, I promised to talk about using structured data with a dataset focused on 1950s Bay Area publishing. To get into that topic, I’m going to talk about 1) setting out with a research question as well as 2) data discovery, and 3) data organization, in order to do 4) initial mapping.
Background to my Research
When I moved to the Bay Area, I (your illustrious Literatures and Digital Humanities Librarian) started exploring UC Berkeley’s collections. I wandered through the Doe Library’s circulating collections and started talking to our Bancroft staff about the special library and archive’s foci. As expected, one of UC Berkeley’s collecting areas is California publishing, with a special emphasis on poetry.
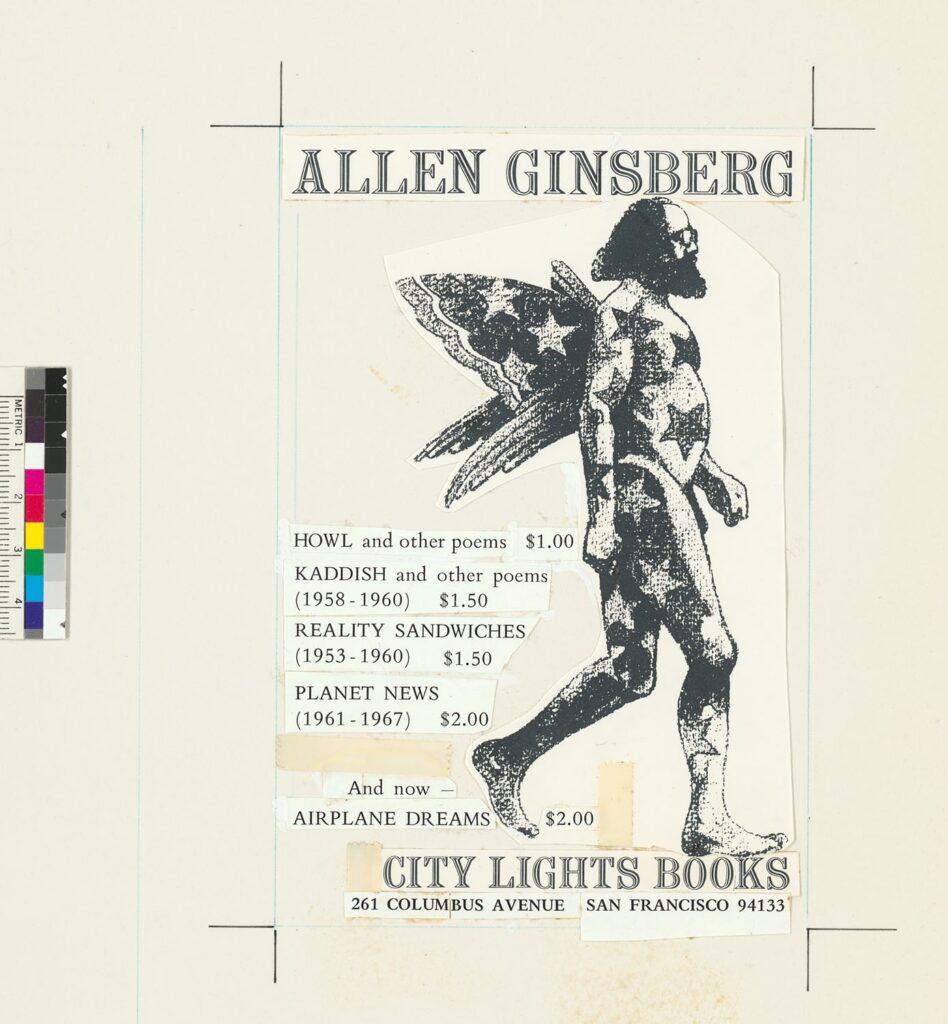
In fact, some of Bancroft’s oft-used materials are the City Light Books collections (link to finding aids in the Online Archive of California) that include some of Allen Ginsberg’s pre-publication drafts of “Howl” and original copies of Howl and Other Poems. You may already know about that poem because you like poetry, or because you watch everything with Daniel Radcliffe in it (IMDB on the 2013 Kill your Darlings). This is, after all, the very poem that led to the seminal trial that influenced U.S. free speech and obscenity laws (often called The Howl Obscenity Trial) . The Bancroft collections have quite a bit about that trial as well as some of Ginsberg’s correspondence with Lawrence Ferlinghetti (poet, bookstore owner, and publisher) during the harrowing legal case. (You can a 2001 discussion with Ferlinghetti on the subject here.)
Research Question
Interested in learning more about Bay Area publishing in general and the period in which Ginsberg’s book was written in particular, I decided to look into the Bay Area publishing environment during the 1950s and now (2020s), starting with the early period. I wanted a better sense of the environment in general as well as public access to books, pamphlets, and other printed material. In particular, I wanted to start with the number of publishers and where they were.
Data Discovery
For a non-digital, late 19th and 20th century era, one of the easiest places to start getting a sense of mainstream businesses is to look in city directories. There was a sweet spot in an era of mass printing and industrialization in which city directories were one of the most reliable sources of this kind of information, as the directory companies were dedicated to finding as much information as possible about what was in different urban areas and where men and businesses were located. The directories, as a guide to finding business, people, and places, were organized in a clear, columned text, highly standardized and structured in order to promote usability.
Raised in an era during which city directories were still a normal thing to have at home, I already knew these fat books existed. Correspondingly, I set forth to find copies of the directories from the 1950s when “Howl” first appeared. If I hadn’t already known, I might have reached out to my librarian to get suggestions (for you, that might be me).
I knew that some of the best places to find material like city directories were usually either a city library or a historical society. I could have gone straight to the San Francisco Public Library’s website to see if they had the directories, but I decided to go to Google (i.e., a giant web index) and search for (historic san francisco city directories). That search took me straight to the SFPL’s San Francisco City Directories Online (link here).
On the site, I selected the volumes I was interested in, starting with Polk’s Directory for 1955-56. The SFPL pages shot me over to the Internet Archive and I downloaded the volumes I wanted from there.
Once the directory was on my computer, I opened it and took a look through the “yellow pages” (i.e., pages with information sorted by business type) for “publishers.”
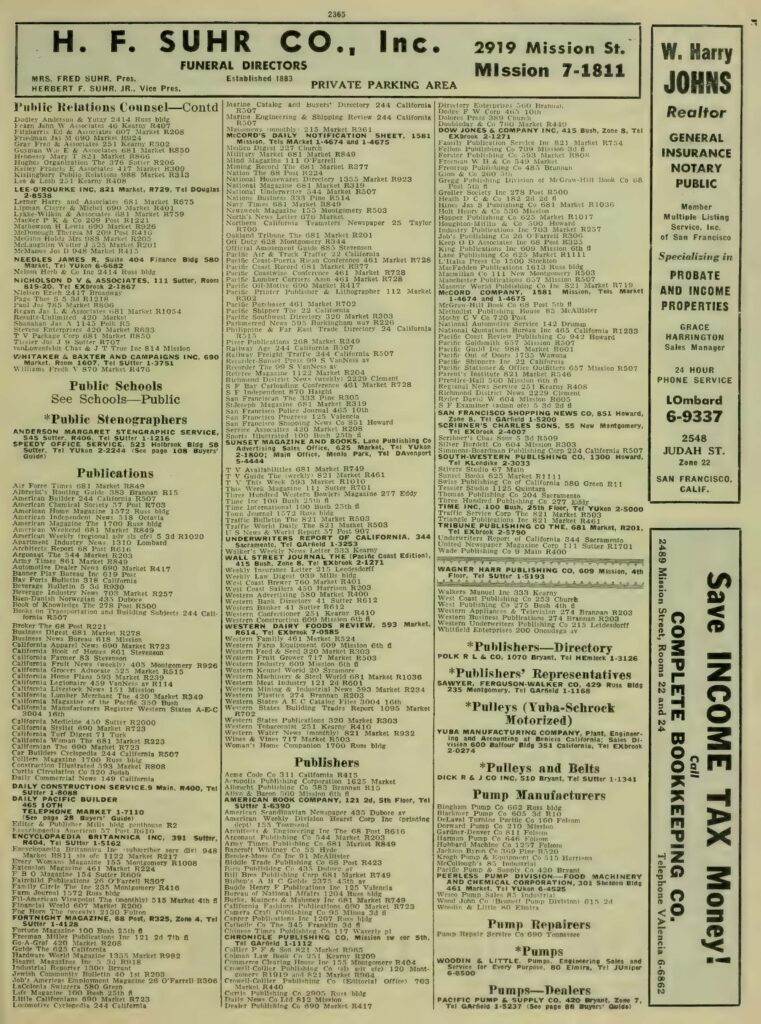
Glancing through the listings, I noted that the records for “publishers” did not list City Light Books. Flipped back to “book sellers,” I found it. That meant that other booksellers could be publishers as well. And, regardless, those booksellers were spaces where an audience could acquire books (shocker!) and therefore relevant. Considering the issue, I also looked at the list for “printers,” in part to capture some of the self-publishing spaces.
I now had three structured lists from one directory with dozens of names. Yet, the distances within the book and inability to reorganize made them difficult to consider together. Furthermore, I couldn’t map them with the structure available in the directory. In order to do what I wanted with them (i.e., meet my research goals), I needed to transform them into a machine readable data set.
Creating a Data Set
Machine Readable
I started by doing a one-to-one copy. I took the three lists published in the directory and ran OCR across them in Adobe Acrobat Professional (UC Berkeley has a subscription; for OA access I recommend Transkribus or Tesseract), and then copied the relevant columns into a Word document.
Data Cleaning
The OCR copy of the list was a horrifying mess with misspellings, cut-off words, Ss understood as 8s, and more. Because this was a relatively small amount of data, I took the time to clean the text manually. Specifically, I corrected typos and then set up the text to work with in Excel (Google Sheets would have also worked) by:
- creating line breaks between entries,
- putting tabs between the name of each institution and corresponding address
Once I’d cleaned the data, I copied the text into Excel. The line breaks functioned to tell Excel where to break rows and the tabs where to understand columns. Meaning:
- Each institution had its own row.
- The names of the institutions and their addresses were in different columns.
Having that information in different spaces would allow me to sort the material either by address or back to its original organization by company name.
Adding Additional Information
I had, however, three different types of institutions—Booksellers, Printers, and Publishers—that I wanted to be able to keep separate. With that in mind, I added a column for EntryType (written as one word because many programs have issues with understanding column headers with spaces) and put the original directory headings into the relevant rows.
Knowing that I also wanted to map the data, I also added a column for “City” and another for “State” as the GIS (i.e., mapping) programs I planned to use wouldn’t automatically know which urban areas I meant. For these, I wrote the name of the city (i.e., “San Francisco”) and then the state (i.e., “California”) in their respective columns and autofilled the information.
Next, for record keeping purposes, I added columns for where I got the information, the page I got it from, and the URL for where I downloaded it. That information simultaneously served for me as a reminder but also as a pointer for anyone else who might want to look at the data and see the source directly.
I put in a column for Org/ID for later, comparative use (I’ll talk more about this one in a further post,) and then added columns for Latitude and Longitude for eventual use.
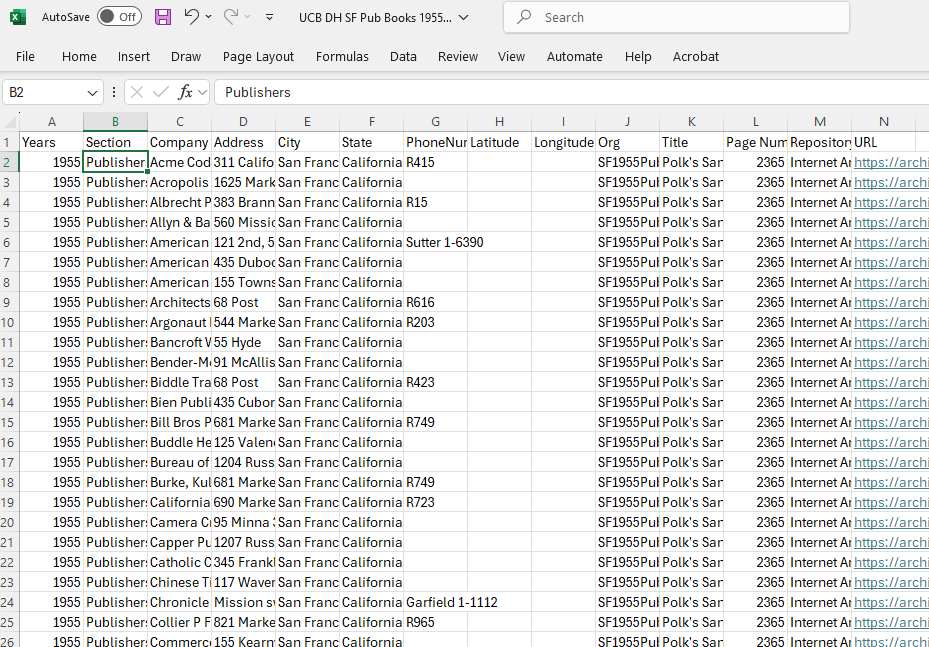
Finally, I saved my data with a filename that I could easily use to find the data again. In this case, I named it “BayAreaPublishers1955.” I made sure to save the data as an Excel file (i.e., .xmlx) and Comma Separated Value file (i.e., .csv) for use and preservation respectively. I also uploaded the file into Google Drive as a Google Sheet so you could look at it.
Initial Mapping of the Data
With that clean dataset, I headed over to Google’s My Maps (mymaps.google.com) to see if my dataset looked good and didn’t show locations in Los Angeles or other spaces. I chose Google Maps for my test because it is one of the easiest GIS programs to use
- because many people are already used to the Google interface
- the program will look up latitude and longitude based on address
- it’s one of the most restrictive, meaning users don’t get overwhelmed with options.
Heading to the My Maps program, I created a “new” map by clicking the “Create a new map” icon in the upper, left hand corner of the interface.
From there, I uploaded my CSV file as a layer. Take a look at the resulting map:
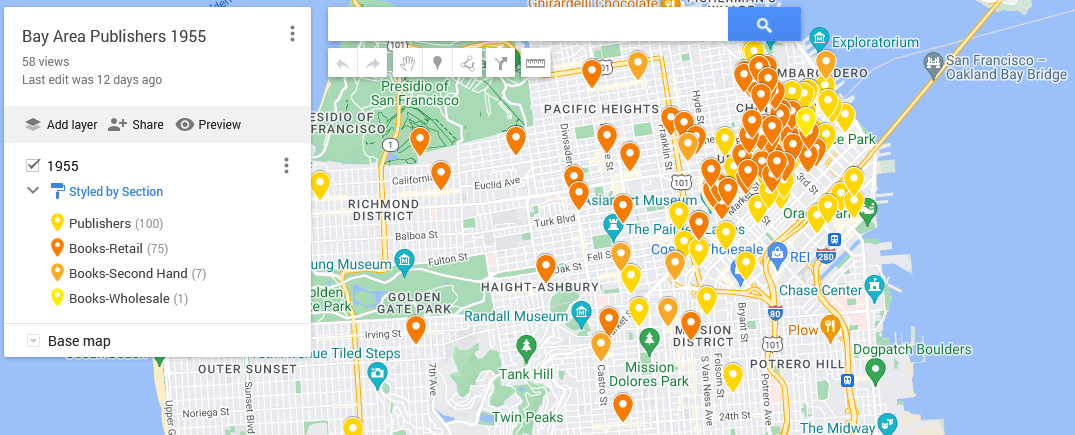
The visualization highlights the centrality of the 1955 San Francisco publishing world, with its concentration of publishing companies and bookstores around Mission Street. Buying books also necessitated going downtown, but once there, there was a world of information at one’s fingertips.
Add in information gleaned from scholarship and other sources about book imports, custom houses, and post offices, and one can start to think about international book trades and how San Francisco was hooked into it.
I’ll talk more about how to use Google’s My Maps in the next post in two weeks!
A&H Data: What even is data in the Arts & Humanities?
This is the first of a multi-part series exploring the idea and use of data in the Arts & Humanities. For more information, check out the UC Berkeley Library’s Data and Digital Scholarship page.
Arts & Humanities researchers work with data constantly. But, what is it?
Part of the trick in talking about “data” in regards to the humanities is that we are already working with it. The books and letters (including the one below) one reads are data, as are the pictures we look at and the videos we watch. In short, arts and humanities researchers are already analyzing data for the essays, articles, and books that they write. Furthermore, the resulting scholarship is data.
For example, the letter below from Bancroft Library’s 1906 San Francisco Earthquake and Fire Digital Collection on Calisphere is data.
George Cooper Pardee, “Aid for San Francisco: Letter from the Mayor in Oregon,”
April 24, 1906, UC Berkeley, Bancroft Library on Calisphere.
One ends up with the question “what isn’t data?”
The broad nature of what “data” is means that instead of asking if something is data, it can be more useful to think about what kind of data one is working with. After all, scholars work with geographic information; metadata (e.g., data about data); publishing statistics; and photographs differently.
Another helpful question is to consider how structured it is. In particular, you should pay attention to whether the data is:
- unstructured
- semi-structured
- structured
The level of structure informs us how to treat the data before we analyze it. If, for example, you have hundreds of of images, you want to work with, it’s likely you’ll have to do significant amount of work before you can analyze your data because most photographs are unstructured.
In contrast, the letter toward the top of this post is semi-structured. It is laid out in a typical, physical letter style with information about who, where, when, and what was involved. Each piece of information, in turn, is placed in standardized locations for easy consumption and analysis. Still, to work with the letter and its fellows online, one would likely want to create a structured counterpart.
Finally, structured data is usually highly organized and, when online, often in machine-readable chart form. Here, for example, are two pages from the Polk San Francisco City Directory from 1955-1956 with a screenshot of the machine-readable chart from a CSV (comma separated value) file below it. This data is clearly structured in both forms. One could argue that they must be as the entire point of a directory is for easy of information access and reading. The latter, however, is the one that we can use in different programs on our computers.
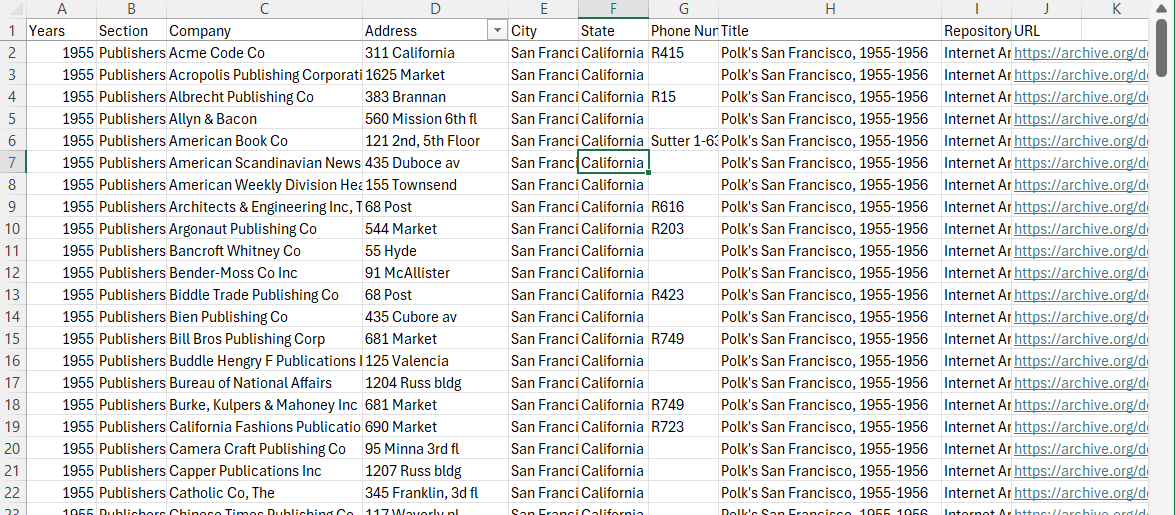
Internet Archive. | Public Domain.
This post has provided a quick look at what data is for the Arts&Humanities.
The next will be looking at what we can do with machine-readable, structured data sets like the publisher’s information. Stay tuned! The post should be up in two weeks.